01
事务基础概念
/**
* Kafka事务基础概念演示
*/
public class KafkaTransactionBasics {
// 1. 事务生产者配置
public static Properties getTransactionalProducerConfig(String transactionalId) {
Properties props = new Properties();
props.put(ProducerConfig.BOOTSTRAP_SERVERS_CONFIG, "localhost:9092");
props.put(ProducerConfig.TRANSACTIONAL_ID_CONFIG, transactionalId);
props.put(ProducerConfig.ENABLE_IDEMPOTENCE_CONFIG, true);
props.put(ProducerConfig.ACKS_CONFIG, "all");
props.put(ProducerConfig.KEY_SERIALIZER_CLASS_CONFIG, StringSerializer.class.getName());
props.put(ProducerConfig.VALUE_SERIALIZER_CLASS_CONFIG, StringSerializer.class.getName());
return props;
}
// 2. 基本事务操作示例
public static void demonstrateBasicTransaction() {
try (KafkaProducer<String, String> producer =
new KafkaProducer<>(getTransactionalProducerConfig("basic-transaction-id"))) {
// 初始化事务
producer.initTransactions();
try {
// 开始事务
producer.beginTransaction();
// 发送多条消息
producer.send(new ProducerRecord<>("topic1", "key1", "value1"));
producer.send(new ProducerRecord<>("topic2", "key2", "value2"));
// 提交事务
producer.commitTransaction();
} catch (Exception e) {
// 回滚事务
producer.abortTransaction();
throw e;
}
}
}
// 3. 事务状态监控
public static class TransactionStateMonitor {
private enum TxState {
INIT, BEGUN, PREPARING_COMMIT, COMMITTED, PREPARING_ABORT, ABORTED
}
private TxState currentState;
private final String transactionalId;
private final long startTime;
public TransactionStateMonitor(String transactionalId) {
this.transactionalId = transactionalId;
this.startTime = System.currentTimeMillis();
this.currentState = TxState.INIT;
}
public void updateState(TxState newState) {
this.currentState = newState;
logStateTransition(newState);
}
private void logStateTransition(TxState newState) {
long duration = System.currentTimeMillis() - startTime;
System.out.printf("Transaction %s moved to state %s after %d ms%n",
transactionalId, newState, duration);
}
}
public static void main(String[] args) {
// 演示基本事务操作
demonstrateBasicTransaction();
}
}
复制
02
事务实现机制
/**
* Kafka事务实现机制详细演示
*/
public class KafkaTransactionImplementation {
// 1. 事务管理器
public static class TransactionManager {
private final KafkaProducer<String, String> producer;
private final TransactionStateMonitor monitor;
private final int maxRetries;
private final long retryBackoffMs;
public TransactionManager(String transactionalId, int maxRetries, long retryBackoffMs) {
Properties props = KafkaTransactionBasics.getTransactionalProducerConfig(transactionalId);
this.producer = new KafkaProducer<>(props);
this.monitor = new TransactionStateMonitor(transactionalId);
this.maxRetries = maxRetries;
this.retryBackoffMs = retryBackoffMs;
// 初始化事务
producer.initTransactions();
}
// 2. 执行事务性操作
public void executeTransactionally(List<ProducerRecord<String, String>> records) {
int attempts = 0;
while (attempts < maxRetries) {
try {
executeTransaction(records);
return;
} catch (Exception e) {
attempts++;
if (attempts == maxRetries) {
throw new RuntimeException("Max retries exceeded", e);
}
sleep(retryBackoffMs * (1L << attempts));
}
}
}
// 3. 具体的事务执行逻辑
private void executeTransaction(List<ProducerRecord<String, String>> records) {
try {
producer.beginTransaction();
monitor.updateState(TransactionStateMonitor.TxState.BEGUN);
// 发送所有消息
for (ProducerRecord<String, String> record : records) {
producer.send(record, (metadata, exception) -> {
if (exception != null) {
throw new RuntimeException("Failed to send record", exception);
}
});
}
monitor.updateState(TransactionStateMonitor.TxState.PREPARING_COMMIT);
producer.commitTransaction();
monitor.updateState(TransactionStateMonitor.TxState.COMMITTED);
} catch (Exception e) {
monitor.updateState(TransactionStateMonitor.TxState.PREPARING_ABORT);
producer.abortTransaction();
monitor.updateState(TransactionStateMonitor.TxState.ABORTED);
throw e;
}
}
// 4. 资源清理
public void close() {
if (producer != null) {
producer.close();
}
}
private void sleep(long ms) {
try {
Thread.sleep(ms);
} catch (InterruptedException e) {
Thread.currentThread().interrupt();
throw new RuntimeException(e);
}
}
}
// 5. 演示使用
public static void main(String[] args) {
TransactionManager manager = new TransactionManager(
"transaction-demo-id", 3, 100);
List<ProducerRecord<String, String>> records = Arrays.asList(
new ProducerRecord<>("topic1", "key1", "value1"),
new ProducerRecord<>("topic2", "key2", "value2")
);
try {
manager.executeTransactionally(records);
} finally {
manager.close();
}
}
}
复制
03
事务消费者实现
/**
* Kafka事务消费者实现示例
*/
public class TransactionalConsumerProducer {
private final KafkaConsumer<String, String> consumer;
private final KafkaProducer<String, String> producer;
private final String consumerGroupId;
private volatile boolean running = true;
public TransactionalConsumerProducer(String transactionalId, String consumerGroupId) {
this.consumerGroupId = consumerGroupId;
// 1. 配置消费者
Properties consumerProps = new Properties();
consumerProps.put(ConsumerConfig.BOOTSTRAP_SERVERS_CONFIG, "localhost:9092");
consumerProps.put(ConsumerConfig.GROUP_ID_CONFIG, consumerGroupId);
consumerProps.put(ConsumerConfig.ISOLATION_LEVEL_CONFIG, "read_committed");
consumerProps.put(ConsumerConfig.ENABLE_AUTO_COMMIT_CONFIG, "false");
consumerProps.put(ConsumerConfig.KEY_DESERIALIZER_CLASS_CONFIG,
StringDeserializer.class.getName());
consumerProps.put(ConsumerConfig.VALUE_DESERIALIZER_CLASS_CONFIG,
StringDeserializer.class.getName());
// 2. 配置生产者
Properties producerProps = new Properties();
producerProps.put(ProducerConfig.BOOTSTRAP_SERVERS_CONFIG, "localhost:9092");
producerProps.put(ProducerConfig.TRANSACTIONAL_ID_CONFIG, transactionalId);
producerProps.put(ProducerConfig.ENABLE_IDEMPOTENCE_CONFIG, true);
producerProps.put(ProducerConfig.KEY_SERIALIZER_CLASS_CONFIG,
StringSerializer.class.getName());
producerProps.put(ProducerConfig.VALUE_SERIALIZER_CLASS_CONFIG,
StringSerializer.class.getName());
this.consumer = new KafkaConsumer<>(consumerProps);
this.producer = new KafkaProducer<>(producerProps);
this.producer.initTransactions();
}
// 3. 消费-处理-生产循环
public void processMessages(String inputTopic, String outputTopic) {
consumer.subscribe(Collections.singletonList(inputTopic));
try {
while (running) {
ConsumerRecords<String, String> records =
consumer.poll(Duration.ofMillis(100));
if (!records.isEmpty()) {
processRecordBatch(records, outputTopic);
}
}
} finally {
closeResources();
}
}
// 4. 处理消息批次
private void processRecordBatch(ConsumerRecords<String, String> records,
String outputTopic) {
try {
producer.beginTransaction();
// 处理每条消息
for (ConsumerRecord<String, String> record : records) {
String processedValue = processRecord(record.value());
ProducerRecord<String, String> outputRecord =
new ProducerRecord<>(outputTopic, record.key(), processedValue);
producer.send(outputRecord);
}
// 提交消费偏移量
Map<TopicPartition, OffsetAndMetadata> offsets = new HashMap<>();
for (TopicPartition partition : records.partitions()) {
List<ConsumerRecord<String, String>> partitionRecords =
records.records(partition);
long lastOffset = partitionRecords.get(partitionRecords.size() - 1)
.offset();
offsets.put(partition, new OffsetAndMetadata(lastOffset + 1));
}
// 在事务中提交偏移量
producer.sendOffsetsToTransaction(offsets,
new ConsumerGroupMetadata(consumerGroupId));
// 提交事务
producer.commitTransaction();
} catch (Exception e) {
producer.abortTransaction();
throw new RuntimeException("Failed to process record batch", e);
}
}
// 5. 业务处理逻辑
private String processRecord(String value) {
// 实现具体的业务处理逻辑
return "Processed: " + value;
}
private void closeResources() {
producer.close();
consumer.close();
}
public void shutdown() {
running = false;
}
}
复制
04
加群请添加作者
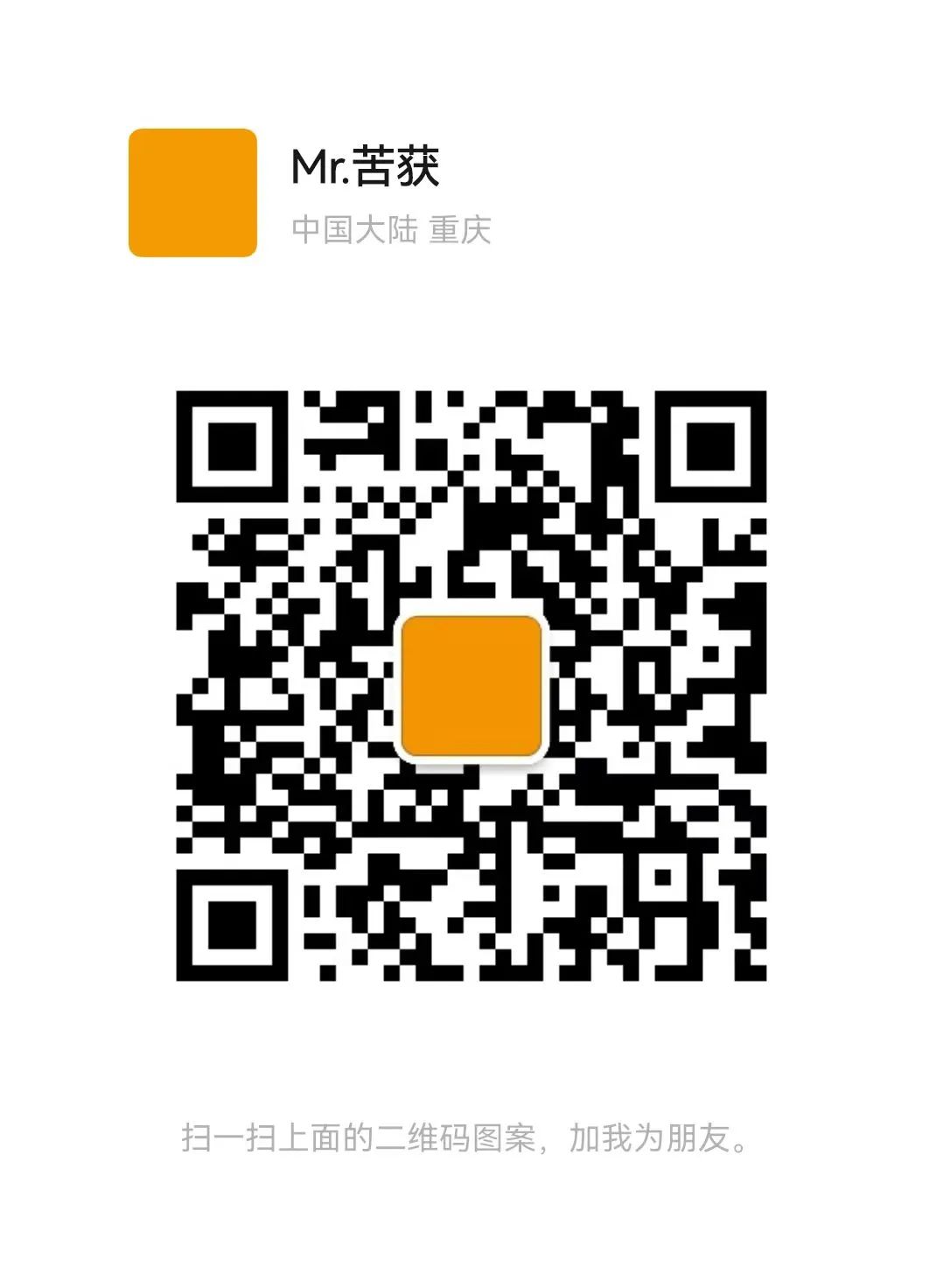
05
获取文档资料
推荐阅读系列文章
建议收藏 | Dinky系列总结篇 建议收藏 | Flink系列总结篇 建议收藏 | Flink CDC 系列总结篇 建议收藏 | Doris实战文章合集 建议收藏 | Paimon 实战文章总结 建议收藏 | Fluss 实战文章总结 建议收藏 | Seatunnel 实战文章系列合集 建议收藏 | 实时离线输数仓(数据湖)总结篇 建议收藏 | 实时离线数仓实战第一阶段总结 超700star!电商项目数据湖建设实战代码 ,拿来即用! 从0到1建设电商项目数据湖实战教程 推荐一套开源电商项目数据湖建设实战代码
如果喜欢 请点个在看分享给身边的朋友
文章转载自大数据技能圈,如果涉嫌侵权,请发送邮件至:contact@modb.pro进行举报,并提供相关证据,一经查实,墨天轮将立刻删除相关内容。