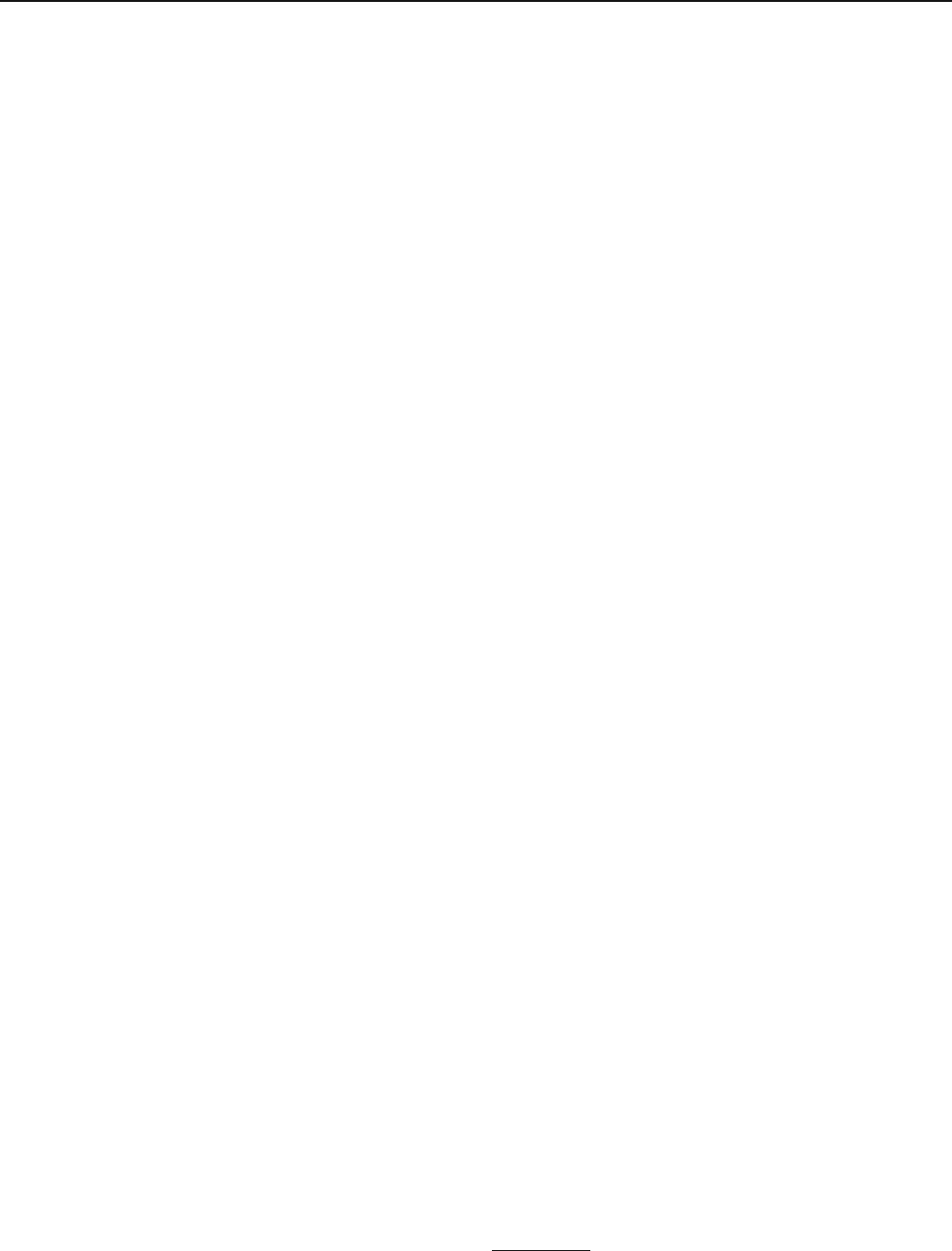
938 K. Zhao et al.
properties prediction and phylogeny construction) [14]. In
addition to supporting applications, subgraph counting can
also be used in a system for query optimization to estimate
the cardinality for complex large joins with cycles, where
a query is to enumerate subgraph matchings in a large data
graph stored in a relational system [2,61].
1.1 Existing approaches and motivation
Exact subgraph counting by subgraph isomorphism is chal-
lenging. As surveyed in [78], there are enumeration meth-
ods [7,10,11,13,42,61,80] and analytical methods [4,60,67,
72]. Full subgraph enumeration is difficult as determining
whether a given query graph exists in a data graph by sub-
graph isomorphism is NP-complete [12,22]. The analytical
approaches do counting by decomposing a query graph into
smaller s ubgraphs, and counting the query graph based on
the counts obtained for the smaller subgraphs. The analyt-
ical methods are designed for some query graphs up to a
certain size (e.g., 5-node query graph), and are difficult to
have a general form for any size of query graphs. Some exact
subgraph counting algorithms, e.g., [60,112] can support
query graphs with up to 6 nodes. Instead of exact subgraph
counting, approximate subgraph counting has been studied
in recent years [15–17,21,41,99], which is designed for small
query graphs over simple unlabeled data graphs. For larger
query graphs, [82] proposes an approximate algorithm that
can count tree-shaped query graphs with up to 12 nodes. [17]
can support approximate subgraph counting for query graphs
with 10 nodes by color coding. These approaches are non-
trivial to be extended to support labeled graphs due to the
intrinsic techniques they use for approximation (e.g., color
coding) [15,17].
The subgraph counting can be supported in
RDBMS
using
SQL
[65,84], multi-way joins [18,52,97,116], and
processed as cardinality estimation. Specifically, consider a
node-labeled undirected graph G = (V , E, L). It can be
stored in an
RDBMS
using a node table, T
V
(V , L), and an
edge table, T
E
(F, T ), where the node table keeps its node-
ids and the labels associated with the node, and the edge table
keeps the edges (node-id pairs). Consider a simple subgraph
counting task to count the number of open triangles among
three nodes, {v
1
,v
2
,v
3
}, which are connected by two edges
(v
1
,v
2
) and (v
2
,v
3
), and the node-labels for v
1
/v
2
/v
3
are
A/B/C, respectively. The subgraph counting by homomor-
phism can be specified as an
SQL
query
selectcount(∗)from
T
V
as T
A
, T
V
as T
B
, T
V
as T
C
, T
E
as T
1
, T
E
as T
2
where T
1
.T = T
2
.F and T
A
.V = T
1
.Fand
T
A
.L =
A
and T
B
.V = T
1
.T and
T
B
.L =
B
and T
C
.V = T
2
.T and T
A
.L =
C
Note that the same subgraph counting by subgraph isomor-
phism needs to add a constraint in the where clause as T
1
.F =
T
2
.T .
1
To process such
SQL
queries, first, the approaches in
[18,65,84,97] decompose a query graph into a set of small
subgraphs, count the subgraph matchings for the smaller sub-
graphs, and aggregate the counts to approximate the final
count. They rely on an assumption that the counts of the
smaller subgraphs are independent, which is impractical for
real large graphs so that it incurs inaccurate estimation. Sec-
ond, the approaches in [52,116] make use of join sampling
that draws matchings from the data graph. These approaches
face drastically increasing sampling failure in the intermedi-
ate join steps when the query graphs and the distribution
of the data graph are complex (i.e., complex topological
structure and label distribution), although the sampling is
independent. The limitations of these approaches cause the
deterioration of estimation accuracy as well as efficiency
on counting f or complex subgraphs. In [69], a benchmark
G-CARE
for cardinality estimation of subgraph matching
(homomorphism) is presented, which investigates estimation
approaches for graphs [21,65,84] as well as relational data
[52,97,116]. With
G-CARE
open source, we verify that join
sampling has its limitation for complex subgraph matching
even by homomorphism when it is used to test for large query
graphs. The difficulty of cardinality estimation comes from
complex data distribution [50].
A question that arises is if machine learning (ML) and deep
learning (DL) can be effectively used for subgraph counting.
Liu et al. in [54] study neural subgraph isomorphism counting
using a dynamic memory network. Their models are trained
on synthetic graphs which are up to 512 nodes (Table 4 in
[54]). Their approach is computationally too expensive to
be used for large data graphs. Below, as subgraph counting
(homomorphism or subgraph isomorphism) can be specified
as an
SQL
query, we discuss if DL approaches studied in
RDBMS
context for approximate query processing (AQP)
or cardinality estimation can be used to support subgraph
counting.
Table 1 summarizes the ML/DL approaches studied for
AQP (the top three) and cardinality estimation (the bottom
twelve). The
SQL
queries they support include join, selec-
tion, and group-by and aggregation. The joins can be either
equi-join, joins between primary-key (PK) and foreign-key
(FK), or precomputed join results. The selection conditions
are on numerical or categorical attributes. For numerical
attributes, the condition can be on a value or a range. For cate-
gorical attributes, string estimation is also considered in [87].
For selection predicates, most approaches support multivari-
ate numerical range predicates and categorical predicates,
and [87,100] support string comparison. For AQP, aggre-
1
Homomorphism allows two nodes in a query graph to be mapped to
the same node in a data graph, where subgraph isomorphism does not.
123
评论