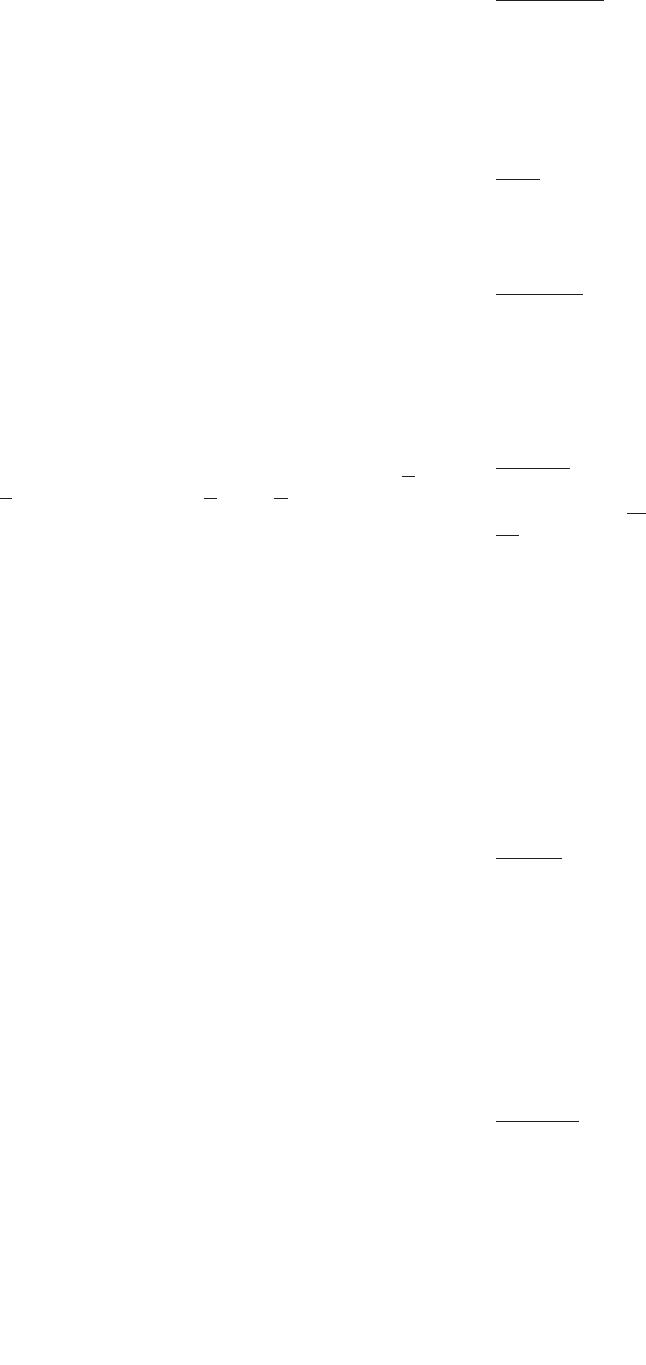
cannot effectively reduce the impact on surrounding vehicles.
To sum up, an ideal decision framework for the autonomous
vehicle can perform safe and comfortable maneuvers with
high driving efficiency and minimal impact on surrounding
vehicles. In particular, reducing the impact plays a vital role
in addressing poor driving behaviors and reducing traffic
congestion or accidents. Intuitively, one can embed a tra-
jectory prediction model [14], [16] in perception modules
(with onboard sensors), which not only capture the current
state of surrounding vehicles, but also proactively anticipate
their future behaviors, and then utilize a deep reinforce-
ment learning-based model to make maneuver decisions [9].
However, the intuition will face two main challenges: (1)
The states of surrounding vehicles are not always observable
due to sensor limitations like detection range and occlusion,
making the trajectory prediction models less effective. (2)
Reinforcement learning-based models struggle to balance the
factors of safety, efficiency, comfort, and impact for complex
vehicle maneuvers, and it is also challenging to measure the
impact factor. In this work, we aim to address the above
challenges and enable the autonomous vehicle to perform
safe and comfortable maneuvers while maximizing its average
velocity and minimizing its impact on surrounding vehicles.
To this end, we proposed a novel perception-and-decision
framework, called HEAD, which consists of an enH
anced
pE
rception module and a mAneuver Decision module. In the
enhanced perception module, we propose a state prediction
model to predict the one-step future states for multiple sur-
rounding vehicles in parallel. To deal with the incomplete
historical states caused by sensor limitations, it first constructs
phantom vehicles based on observable surrounding vehicles
and organizes their relationships using spatial-temporal graph,
and then utilizes a graph attention mechanism with an LSTM
to enable vehicle interactions and parallel prediction. For the
maneuver decision module, it first receives the future states of
surrounding vehicles and formulates the maneuver decision
task as a Parameterized Action Markov Decision Process
(PAMDP) with discrete lane change behaviors and continuous
velocity change behavior, and then uses a deep reinforcement
learning-based model and a properly designed reward function
to solve the PAMDP, which learn an optimized policy for the
autonomous vehicle to achieve our objective. In summary, we
make the following contributions:
• We develop a perception-and-decision framework that en-
ables the autonomous vehicle to perform safe, efficient, and
comfortable maneuvers with minimal impact on other vehicles.
• We propose a graph-based state prediction model with
a strategy of phantom vehicle construction to solve sensor
limitations and support high-accuracy prediction in parallel.
• We propose a deep reinforcement learning-based model
and a hybrid reward function to make maneuver decisions in
continuous action space that follows a parameterized action
Markov decision process.
• We conduct extensive experiments to evaluate HEAD on
real and simulated data, verifying the effectiveness on both
macroscopic and microcosmic metrics.
II. O
VERVIEW
A. Preliminary Concepts
Environment.
We consider an interactive environment where
there are one autonomous vehicle A and a set of conventional
vehicles C traveling on a straight multi-lane road. For the sake
of simplicity, parking and turning are not considered for now.
The autonomous vehicle can obtain the states (i.e., locations
and velocities) of surrounding conventional vehicles through
its sensors, and perform a maneuver at each time instant t
within a target time duration T of interest.
Lane.
A lane is part of the road used to guide vehicles
in the same direction. Herein, all the lanes are numbered
incrementally from the leftmost side to the rightmost side,
i.e., l
1
,l
2
,...,l
κ
, where l
1
and l
κ
indicate the leftmost lane
and rightmost lane, respectively.
Time Step.
In order to model the problem more concisely,
we treat the continuous time duration as a set of discrete
time steps, i.e., T = {1, 2,...,t,...}. We denote Δt as
the time interval between two consecutive time steps, which
serves as the minimum frequency for the autonomous vehicle
to perform maneuvers. Following the settings used in the
previous work [14], [17], the time granularity in this work
is set to 0.5 seconds (i.e., Δt =0.5s).
Location.
(C
t
i
.lat, C
t
i
.lon) and (A
t
.lat, A
t
.lon) indicate the
locations of C
i
and A, respectively, at time step t, where
lat denotes the lat
eral lane number and lon refers to the
lon
gitudinal location of a vehicle traveled from the origin.
d
lon
(C
t
i
,A
t
) denotes the relative longitudinal distance be-
tween C
i
and A at time step t, which can be calculated as
follows:
d
lon
(C
t
i
,A
t
)=C
t
i
.lon − A
t
.lon (1)
In addition, the d
lat
(C
t
i
,A
t
) denotes the relative lateral dis-
tance between C
i
and A at time step t, which can be calculated
as follows:
d
lat
(C
t
i
,A
t
)=(C
t
i
.lat − A
t
.lat) ∗ wid
l
(2)
where wid
l
is the width of a lane. An advantage of using
this type of lane-aware location is to allow us to focus on the
lane change behavior itself without worrying about the lateral
location of the vehicle.
Velocity.
C
t
i
.v and A
t
.v indicate the longitudinal velocities of
C
i
and A, respectively, at time step t. v(C
t
i
,A
t
) denotes the
relative longitudinal velocity between C
i
and A at time step
t, which can be calculated as follows:
v(C
t
i
,A
t
)=C
t
i
.v − A
t
.v (3)
Benefiting from the discrete time step and the lane-aware
location, the lateral motion between two consecutive time
steps is assumed to be the uniform motion [14], [18], so
we focus on the longitudinal velocity in this work. In the
rest of the paper, we use velocity and longitudinal velocity
interchangeably when no ambiguity is caused.
Maneuver.
A maneuver is a pair of a lateral lane change
behavior and a longitudinal velocity change behavior simul-
taneously performed by a vehicle [19]. (A
t
.b, A
t
.a) represents
3256
Authorized licensed use limited to: Huawei Technologies Co Ltd. Downloaded on August 23,2024 at 09:42:07 UTC from IEEE Xplore. Restrictions apply.
评论