传统 RAG(检索增强生成)通常使用向量数据库检索相关文档来提高大模型的问题回答准确性,但是基于向量的 RAG 不能够很好的表达事物之间的联系。而GraphRAG基于知识图谱的检索增强生成,能够提供结构化检索能力,将图作为传统的RAG多路召回的一环,使知识表达更易解释、对复杂关系更易支持。
目前 openGauss AGEGraph 已经提供了图数据库引擎的能力。本文将详细介绍如何借助大模型以及 openGauss 图数据库快速提取文档知识图谱并持久化。 随后,将问题输入大模型,大模型自动提取关键词并转换为图查询语句连接 openGauss 图数据库进行图数据检索。最后,将图数据输入大模型生成问题答案反馈给用户。
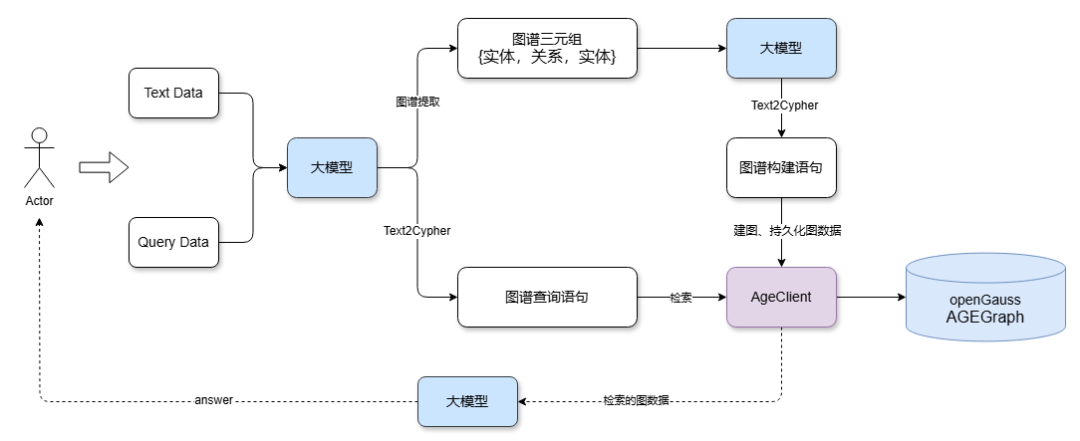
1.环境准备
1.1 openGauss 容器化部署
可参考openGauss容器镜像安装部署openGauss。
openGauss容器启动后可以执行docker ps
查看容器是否在运行 输出
CONTAINER ID IMAGE COMMAND CREATED STATUS PORTS NAMES
253714c9c869 opengauss:7.0.0-rc1 "entrypoint.sh gauss…" 8 minutes ago Up 8 minutes 0.0.0.0:6543->5432/tcp opengauss-age
复制
1.2 AGE插件安装
#进入openGauss容器中 253714c9c869为 CONTAINER ID
docker exec -it 253714c9c869 bash
#切换到omm用户
su omm
#omm用户,连接数据库,默认使用omm数据库
gsql -r
#连接数据库后,omm数据库安装age插件
create extension age;
#退出数据库连接
\q
复制
1.3 python依赖安装
pip install -U langchain_community langchain langgraph langchain-ollama langchain-experimental langchain-openai
复制
下载已适配的 langchain_community 仓库,操作如下:
1.3.1 下载适配好的langchain_community仓
git clone https://gitee.com/lin-qiang123/langchain.git
复制
1.3.2 查找pip 安装的langchain_community位置
pip show langchain_community
复制
Name: langchain-community
Version: 0.3.20
Summary: Community contributed LangChain integrations.
Home-page:
Author:
Author-email:
License: MIT
Location: c:\users\test\appdata\local\programs\python\python310\lib\site-packages #依赖库安装的位置
Requires: numpy, PyYAML, aiohttp, tenacity, dataclasses-json, langchain, httpx-sse, SQLAlchemy, pydantic-settings, langchain-core, langsmith, requests
Required-by: langchain-experimental
复制
1.3.3 替换langchain-community
在git clone 下来的代码仓中,找到langchain\libs\community\langchain_community文件夹,复制并且覆盖到Location
显示的文件夹中
2. 利用 模型和 openGauss AGEGraph 快速构建知识图谱和检索
本文使用百炼大模型,取得api-key,并且配置DASHSCOPE_API_KEY环境变量
2.1 初始化大模型
from langchain_community.llms import Tongyi
import os
os.environ["DASHSCOPE_API_KEY"] = "sk-**"
graph_llm =Tongyi(model="qwen-plus", temperature=0, base_url="https://dashscope.aliyuncs.com/compatible-mode/v1")
复制
2.2 通过大模型提取文本数据中的实体和关系
from langchain_core.documents import Document
from langchain_experimental.graph_transformers import LLMGraphTransformer
#设置需要提取的实体类型和关系
llm_transformer = LLMGraphTransformer(
llm=graph_llm,
allowed_nodes=["Person", "Organization", "Location", "Award", "ResearchField"],
allowed_relationships = ["SPOUSE", "AWARD", "FIELD_OF_RESEARCH", "WORKS_AT", "IN_LOCATION"],
)
text = """
Marie Curie, 7 November 1867 – 4 July 1934, was a Polish and naturalised-French physicist and chemist who conducted pioneering research on radioactivity.
She was the first woman to win a Nobel Prize, the first person to win a Nobel Prize twice, and the only person to win a Nobel Prize in two scientific fields.
Her husband, Pierre Curie, was a co-winner of her first Nobel Prize, making them the first-ever married couple to win the Nobel Prize and launching the Curie family legacy of five Nobel Prizes.
She was, in 1906, the first woman to become a professor at the University of Paris.
Also, Robin Williams.
"""
# 将文本转化为图
documents = [Document(page_content=text)]
graph_documents = llm_transformer.convert_to_graph_documents(documents)
print(f"Nodes from graph doc:{graph_documents[0].nodes}")
print(f"Relationships from graph doc:{graph_documents[0].relationships}")
复制
Nodes from graph doc:[Node(id='Nobel Prize', type='Award', properties={}), Node(id='University of Paris', type='Organization', properties={}), Node(id='Marie Curie', type='Person', properties={}), Node(id='radioactivity', type='ResearchField', properties={}), Node(id='Pierre Curie', type='Person', properties={})]
Relationships from graph doc:[Relationship(source=Node(id='Marie Curie', type='Person', properties={}), target=Node(id='radioactivity', type='ResearchField', properties={}), type='FIELD_OF_RESEARCH', properties={}), Relationship(source=Node(id='Marie Curie', type='Person', properties={}), target=Node(id='Nobel Prize', type='Award', properties={}), type='AWARD', properties={}), Relationship(source=Node(id='Marie Curie', type='Person', properties={}), target=Node(id='Nobel Prize', type='Award', properties={}), type='AWARD', properties={}), Relationship(source=Node(id='Marie Curie', type='Person', properties={}), target=Node(id='Pierre Curie', type='Person', properties={}), type='SPOUSE', properties={}), Relationship(source=Node(id='Pierre Curie', type='Person', properties={}), target=Node(id='Nobel Prize', type='Award', properties={}), type='AWARD', properties={}), Relationship(source=Node(id='Marie Curie', type='Person', properties={}), target=Node(id='University of Paris', type='Organization', properties={}), type='WORKS_AT', properties={})]
复制
2.3 实例化 openGauss AGEGraph 客户端,持久化图数据
from langchain_community.graphs.age_graph import AGEGraph
conf = {
"database": "omm",
"user": "gaussdb",
"password": "YourPassoword",
"host": "Your IP",
"port": 8888,
"sslmode": "disable"
}
graph=AGEGraph(graph_name='graph_test1',conf=conf,create=True)
graph.add_graph_documents(graph_documents)
graph.refresh_schema()
复制
2.4 大模型 Text2Cypher:提取问题关键词并生成图检索语句
通过增加提示词cypher_prompt
,可以让大模型生成的图查询语句更加精准
from langchain_core.prompts import PromptTemplate
from langchain.chains import GraphCypherQAChain
cypher_prompt = PromptTemplate(
template="""你是 AGE Cypher 查询生成的专家。
使用以下架构生成一个 Cypher 查询,以回答给定问题。
不要出现name和properties和cypher
架构:
{schema}
问题:{question}
Cypher 查询:""",
input_variables=["schema", "question"],
)
chain = GraphCypherQAChain.from_llm(
graph_llm, graph=graph, verbose=True, allow_dangerous_requests=True, cypher_validation=True, return_intermediate_steps=True,cypher_prompt=cypher_prompt
)
question = "Who get Nobel Prize ?"
result = chain.invoke({"query": question})
复制
输出
Entering new GraphCypherQAChain chain… Generated Cypher: MATCH (p:Person)-[:AWARD]->(a:Award) WHERE a.id = “Nobel Prize” RETURN p.id Full Context: [{'p_id': 'Pierre Curie'}, {'p_id': 'Marie Curie'}]
3. 大模型依据检索的图数据生成回答
3.1 设置提示词
prompt = PromptTemplate(
template="""You are an assistant for question-answering tasks.
Use the following pieces of retrieved context from a graph database to answer the question. If you don't know the answer, just say that you don't know.
Use two sentences maximum and keep the answer concise:
Question: {question}
Graph Context: {graph_context}
Answer:
""",
input_variables=["question", "graph_context"],
)
复制
3.2 生成回答
from langchain_core.output_parsers import StrOutputParser
composite_chain = prompt | graph_llm |StrOutputParser()
answer = composite_chain.invoke(
{"question": question, "graph_context": result}
)
print(answer)
复制
Marie Curie and Pierre Curie received the Nobel Prize. They were recognized for their groundbreaking work in radioactivaity.
复制
4. 总结
结合大模型和 openGauss AGEGraph 图数据库,我们可以快速进行知识图谱的生成、持久化以及检索,完成 GraphRAG 的搭建。此外,当前 openGauss 还支持标量、向量类型存储与检索,只需部署 openGauss 数据库,即可实现支持标量、向量、知识图谱多路召回的更强 RAG 系统。