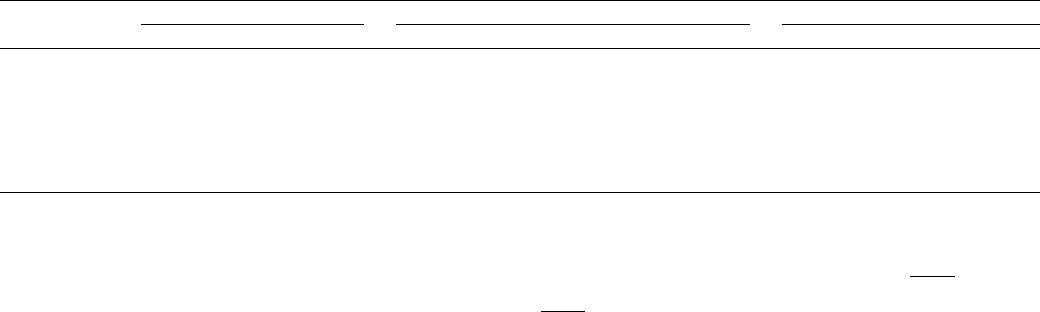
G. Kang, S. Chen and H. Li BenchCouncil Transactions on Benchmarks, Standards and Evaluations 3 (2023) 100122
Table 1
The key designs of HTAP databases: can HTAP benchmarks evaluate them?
Benchmark name Performance isolation Real-time analytics Component performance
OLTP workloads OLAP workloads Fresh data generation rate Fresh data access granularity Index mechanism Query range control
CH-benCHmark
√ √
HTAPBench
√ √
CBTR
√ √
OLxPBench
√ √
HATtrick
√ √
ADAPT
√ √ √
HAP
√ √ √
Micro-benchmark
√ √ √ √ √ √
into column-format data to decrease data movement. In contrast to
TiDB, which deploys the row-based and column-based data stores on
separate data nodes, some HTAP databases [9,14–17] deploy the row-
based and column-based data stores on the same server to prevent data
update propagation across the different data nodes. It slows down the
latency of delta updates moving but poses a significant challenge to
performance isolation.
Equally as important as it is to track advanced technologies for
HTAP databases is to evaluate these HTAP databases. HTAP bench-
marks must measure how well the HTAP databases can do performance
isolation and real-time analytics. We will introduce the existing HTAP
benchmarks from schema design, workload composition, and metrics
as shown in Table 1.
Firstly, there are stitched schema and semantically consistent sch-
ema. The stitched schema is combined with the TPC-C schema [18] and
TPC-H [19] schema. It extracts the New-Order, Stock, Customer, Order-
line, Orders, Item, Warehouse, District, and History relationships from
TPC-C schema [18] to integrate them with the Supplier, Country, and
Region relationships of TPC-H schema [19]. CH-benCHmark [20] pro-
poses the stitched schema, which is followed by HTAPBench [21] and
Swarm64 [22]. Analytical queries cannot access the valuable data gen-
erated by online transactions and stored in the History table when using
the stitched schema. And the stitched schema will affect the semantics
of HTAP benchmarks. Therefore, OLxPBench [23] advocates that HTAP
benchmarks should employ the semantically consistent schema instead
of the stitched schema. The semantically consistent schema emphasizes
that online transactions and analytical queries access the same schema.
Analytical queries can access all business data generated by online
transactions. The semantically consistent schema can thus reveal the
performance inference between OLTP and OLAP workloads. CBTR [24,
25], OLxPBench [23], HATtrick [26], ADAPT [27], and HAP [28]
benchmark all employ semantically consistent schema described in
Sections 5 and 6.
Secondly, HTAP benchmarks include OLTP workloads, OLAP work-
loads, and hybrid workloads. OLTP workloads combine read and write
operations, whereas OLAP workloads are read-intensive. Hybrid work-
load refers to the analytical query performed between online transac-
tions. Existing HTAP benchmarks include OLTP and OLAP workloads
to investigate performance inference between them. OLxPBench is the
only benchmark that evaluates the true HTAP capability of HTAP
databases using hybrid workloads. Complex online transactions and
analytical queries have a lot of operations, so it is hard to judge how
well each operation works on its own. ADAPT [27] and HAP [28]
are Micro-benchmarks for a specific operation. However, the ADAPT
and HAP benchmarks only include a handful of typical HTAP work-
loads. ADAPT and HAP, for instance, include an insufficient number of
scan queries to evaluate index performance. Micro-benchmarks should
provide point scans, small-range and large-range queries for HTAP
database evaluation. There are a few Micro-benchmarks available for
HTAP databases.
Thirdly, the metrics of HTAP databases are separated into two
categories: throughput metrics and latency metrics. The HTAP database
evaluates the throughput of OLTP workloads using the transactions per
second (tps) and transactions per minute (tpmC) metrics. The HTAP
database evaluates the throughput of OLAP workloads using the queries
completed per second (qps) and queries completed per hour (QphH)
metrics. CH-benCHmark [20] proposes the metrics
𝑡𝑝𝑚𝐶
𝑄𝑝ℎ𝐻
@𝑡𝑝𝑚𝐶 and
𝑡𝑝𝑚𝐶
𝑄𝑝ℎ𝐻
@𝑄𝑝ℎ𝐻 for evaluating the performance isolation between OLTP
and OLAP workloads. The former metric considers online transactions
the primary workload, while the latter considers analytical queries
the primary workload. In contrast, Anja Bog et al. [26]. establish
the HATtrick benchmark, which equalizes transactional and analytical
workloads. HATtrick [26] defines the throughput frontier and freshness
metrics for measuring performance isolation and data freshness, as
specified in Section 5.5. HTAP benchmarks utilize average latency and
tail latency metrics in addition to throughput metrics. Average latency
is the average time it takes for a transaction/query to be processed,
whereas tail latency refers to the high percentile latency. Tail latency
is an important metric to consider in HTAP databases where a small
number of lengthy transactions/queries can substantially impact overall
performance or user experience.
This paper makes the following contributions. (1) We systematically
introduce the advanced technologies adopted by HTAP databases for
these key designs; (2) We summarize the pros and cons of the state-
of-the-art and state-of-the-practice HTAP benchmarks for key designs
of HTAP databases; (3) We quantitatively compared the differences
between micro-benchmarks and macro-benchmarks in evaluating the
real-time analytical capabilities of HTAP databases. Micro-benchmark
can control the generation and access granularity of fresh data, en-
abling precise measurement of real-time analytical capabilities of HTAP
databases. (4) We measure the performance of individual components
of the HTAP database, such as the indexing mechanism. By isolating
specific operations, developers can test the performance of these com-
ponents under different workloads and configurations, which is the
foundation of component optimization.
2. Motivation — Micro-benchmarks can control the rate at which
fresh data is generated and the granularity of access, which dis-
tinguishes them from macro-benchmarks
HTAP databases are extreme lack the micro-benchmark because
there is no open-source micro-benchmark. We design and implement
a micro-benchmark to investigate the distinction between the micro-
benchmark and the macro-benchmark. We select the state-of-the-art
HTAP benchmark OLxPBench as the micro-benchmark comparison ob-
ject. Micro-benchmark is better suited for real-time analytics evaluation
because it precisely controls the rate at which fresh data is generated
and the granularity of fresh data access. Micro-benchmark queries
typically consist of a single statement. For instance, the analytical query
calculates the number of rows within a specified range. This indicates
that the computational intensity of analytical queries can be managed
by adjusting their computational range. And the transactional query
updates the value of the specified column in a random row.
Micro-benchmark can adjust the rate at which fresh data is gener-
ated to assess the performance of data update propagation between the
transactional and analytical instances. The performance interference
between transactional and analytical queries can be disregarded when
2
相关文档
评论