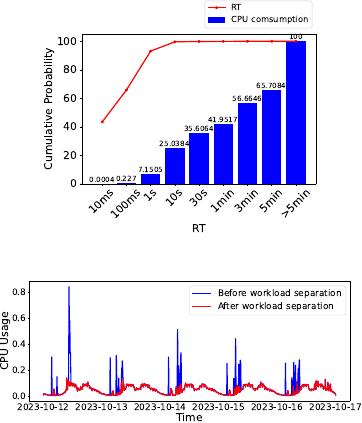
SIGMOD-Companion ’24, June 9–15, 2024, Santiago, AA, Chile Wei Li et al.
Figure 1: Query workloads from an AnalyticDB instance.
Figure 2: CPU usage over a ve-day period.
for short-running queries. A more advanced strategy, termed work-
load isolation, is showcased in Figure 3 (b). Systems like IBM DB2 [
13
],
Microsoft SQL Server [25], and Teradata [38] adopt this approach,
enabling the segregation of queries into multiple queues with des-
ignated resource caps and concurrency limits. Approaches such as
Auto-WLM [
33
] have introduced auto-scaling into cloud data ware-
houses. As demonstrated in Figure 3 (c), auto-scaling dynamically
adjusts the size of a multi-cluster group, such as Snowake’s multi-
cluster warehouses [
10
,
14
,
24
] and Redshift’s concurrency scaling
clusters [
33
] in response to workload uctuations. Furthermore, to
facilitate ecient resource provisioning across multiple database
instances, cloud providers often manage a shared resource pool,
such as Twine [37] and Eigen [19], serving as prime examples.
Despite the signicant evolution of workload management and
auto-scaling in cloud data warehouses, to the best of our knowledge,
no existing solutions have addressed all the challenges below:
First, the execution of mixed heterogeneous workloads leads to
poor performance of long-running queries due to:
C1
Cooperative multi-tasking query execution. The preva-
lent cooperative multi-tasking model [
18
,
35
] in cloud data
warehouses, which limits query tasks to one-second thread
execution, boosts short-running query performance at the
expense of long-running query eciency.
C2
Complex concurrency control. Given the skewed distri-
bution of query types (Figure 1), workload managers that
prioritize short-running queries often diminish the perfor-
mance of long-running ones.
And the auto-scaling of mixed heterogeneous workloads on multi-
cluster groups is facing the following challenge:
C3
Stranded resources caused by workload spikes. Proac-
tive auto-scaling depends on precise time series forecasting
to anticipate workload demands accurately. Existing method-
ologies like Autopilot [
30
], P-Store [
36
], and Eigen [
19
] of-
ten fall short in predicting workload spikes, such as those
caused by ad-hoc queries. To solve this problem, these sys-
tems typically implement a buer strategy, allocating addi-
tional resource margins. However, the cost of the margins is
non-negligible (i.e., stranded resources).
To address the challenges, we present Flux, a cloud-native work-
load auto-scaling platform for Alibaba AnalyticDB. As shown in
Figure 3 (d), Flux implements a pioneering de coupled auto-scaling
architecture which addresses the challenges associated with mixed
execution workloads (C1 and C2) and resource ineciency dur-
ing spikes (C3). Flux separates the management of short-running
and long-running queries to enhance overall system performance.
For short-running queries, Flux adeptly adjusts the multi-cluster
group’s size by either scaling in/out, relying on proactive work-
load forecasting algorithms. Long-running queries, characterized
by their substantial resource demands and high elasticity toler-
ance, are managed distinctly. Flux dynamically allocates resources
by constructing and scaling clusters up or down as required, and
then promptly releases these resources once the query execution is
complete. Figure 2 illustrates that, following the separation of long-
running queries, the CPU usage associated with short-running
queries (red line) exhibits greater seasonality and predictability,
making it more amenable to accurate forecasting.
To fulll the objectives, we rst created a query dispatcher
equipped with a SQL template classier and a machine learning
(ML) classier to identify short-running and long-running queries.
Short-running queries are sent to a multi-cluster group for prompt
processing, while long-running queries are assigned to dedicated
per-job clusters. Second, we engineered a multi-cluster auto-scaler
for short-running queries and a job resource scheduler for long-
running queries that independently scale/schedule resources.
In addition, to reduce stranded resources in the shared resource
pool (C3), we leverage the emerging serverless container instance
services, such as Alibaba ECI [
8
] and AWS Fargate [
2
]. These ser-
vices enable rapid resource provisioning and oer a serverless pric-
ing model, thus facilitating the creation of an on-demand resource
pool. While this on-demand pool provides exibility, it comes with
a higher per-hour cost. To address this, we have constructed a cost
model that operates across both the shared and on-demand resource
pools. Utilizing this model, we devised innovative auto-scaling al-
gorithms aimed at minimizing the overall resource cost.
Our contributions include the following:
•
Architecture: We introduce the architecture of Flux, a pi-
oneering decoupled auto-scaling architecture. This novel
architecture addresses challenges C1-C3 by enhancing query
performance and the resource utilization ratio.
•
Resource Cost Model: We develop a cost model that inte-
grates shared and on-demand resource pools, utilizing Al-
ibaba ECI services. Our model aims to minimize resource
costs for cloud vendors, ensuring economic operation with-
out sacricing service quality.
•
Empirical Evaluation: We evaluate Flux using both public
benchmarks and production clusters with real-world work-
loads. Our results demonstrate substantial improvements
over existing methods: query response time (RT) is reduced
by up to 75%, resource utilization ratio is increased by 19.0%,
and the cost of stranded resources is cut by 77.8%.
评论