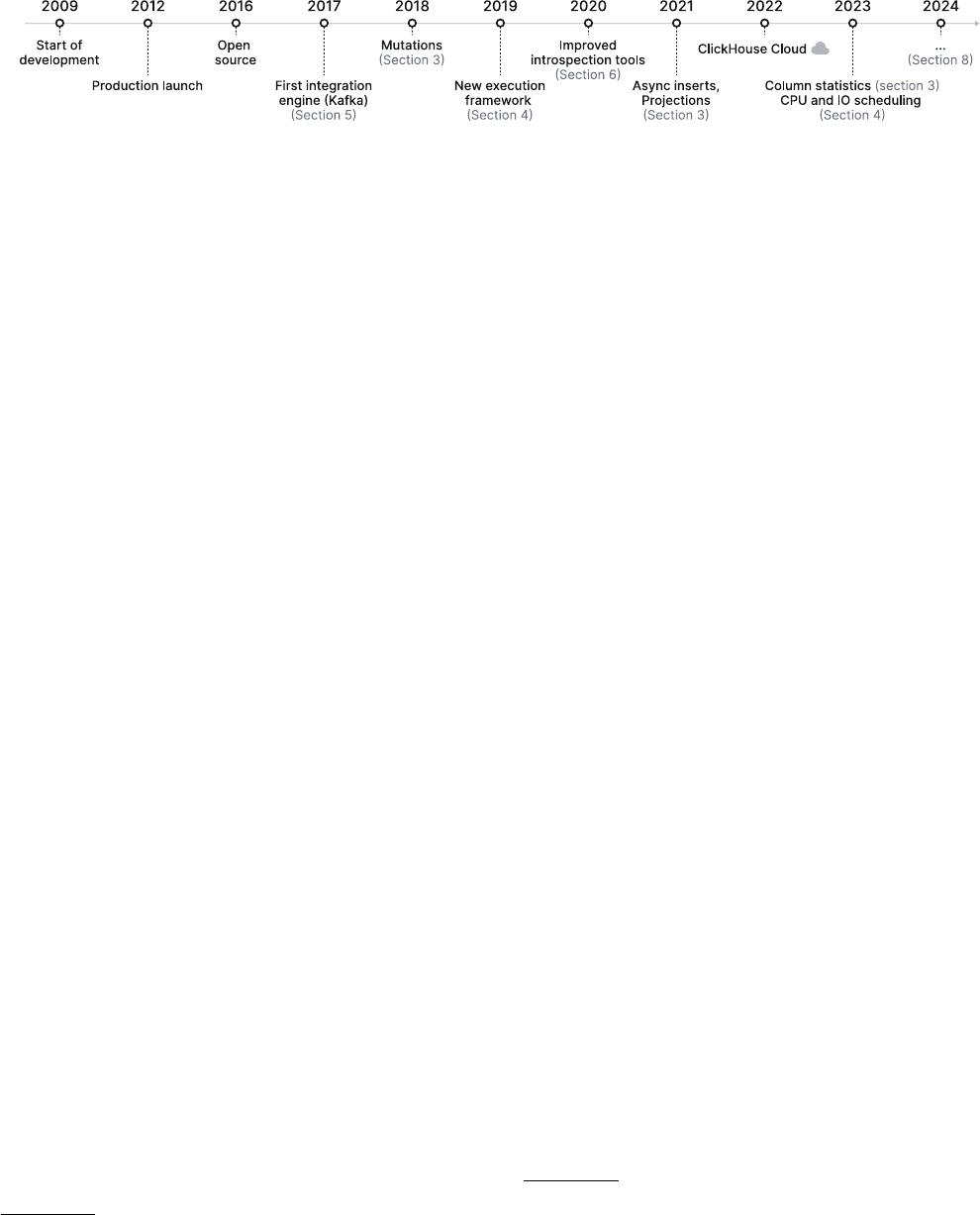
Figure 1: ClickHouse timeline.
2 ARCHITECTURE
As shown by Figure 2, the ClickHouse engine is split into three main
layers: the query processing layer (described in Section 4), the stor-
age layer (Section 3), and the integration layer (Section 5). Besides
these, an access layer manages user sessions and communication
with applications via dierent protocols. There are orthogonal com-
ponents for threading, caching, role-based access control, backups,
and continuous monitoring. ClickHouse is built in C++ as a single,
statically-linked binary without dependencies.
Query processing follows the traditional paradigm of parsing in-
coming queries, building and optimizing logical and physical query
plans, and execution. ClickHouse uses a vectorized execution model
similar to MonetDB/X100 [
11
], in combination with opportunistic
code compilation [
53
]. Queries can be written in a feature-rich SQL
dialect, PRQL [76], or Kusto’s KQL [50].
The storage layer consists of dierent table engines that encap-
sulate the format and location of table data. Table engines fall into
three categories: The rst category is the MergeTree* family of
table engines which represent the primary persistence format in
ClickHouse. Based on the idea of LSM trees [
60
], tables are split
into horizontal, sorted parts, which are continuously merged by
a background process. Individual MergeTree* table engines dier
in the way the merge combines the rows from its input parts. For
example, rows can be aggregated or replaced, if outdated.
The second category are special-purpose table engines, which
are used to speed up or distribute query execution. This category
includes in-memory key-value table engines called dictionaries. A
dictionary caches the result of a query periodically executed against
an internal or external data source. This signicantly reduces ac-
cess latencies in scenarios, where a degree of data staleness can
be tolerated.
2
Other examples of special-purpose table engines in-
clude a pure in-memory engine used for temporary tables and the
Distributed table engine for transparent data sharding (see below).
The third category of table engines are virtual table engines for
bidirectional data exchange with external systems such as relational
databases (e.g. PostgreSQL, MySQL), publish/subscribe systems (e.g.
Kafka, RabbitMQ [
24
]), or key/value stores (e.g. Redis). Virtual
engines can also interact with data lakes (e.g. Iceberg, DeltaLake,
Hudi [36]) or les in object storage (e.g. AWS S3, Google GCP).
ClickHouse supports sharding and replication of tables across
multiple cluster nodes for scalability and availability. Sharding par-
titions a table into a set of table shards according to a sharding
expression. The individual shards are mutually independent ta-
bles and typically located on dierent nodes. Clients can read and
write shards directly, i.e. treat them as separate tables, or use the
Distributed special table engine, which provides a global view of
2
Blog post: clickhou.se/dictionaries
all table shards. The main purpose of sharding is to process data
sets which exceed the capacity of individual nodes (typically, a few
dozens terabytes of data). Another use of sharding is to distribute
the read-write load for a table over multiple nodes, i.e., load balanc-
ing. Orthogonal to that, a shard can be replicated across multiple
nodes for tolerance against node failures. To that end, each Merge-
Tree* table engine has a corresponding ReplicatedMergeTree* engine
which uses a multi-master coordination scheme based on Raft con-
sensus [
59
] (implemented by Keeper
3
, a drop-in replacement for
Apache Zookeeper written in C++) to guarantee that every shard
has, at all times, a congurable number of replicas. Section 3.6 dis-
cusses the replication mechanism in detail. As an example, Figure 2
shows a table with two shards, each replicated to two nodes.
Finally, the ClickHouse database engine can be operated in on-
premise, cloud, standalone, or in-process modes. In the on-premise
mode, users set up ClickHouse locally as a single server or multi-
node cluster with sharding and/or replication. Clients communi-
cate with the database over the native, MySQL’s, PostgreSQL’s
binary wire protocols, or an HTTP REST API. The cloud mode is
represented by ClickHouse Cloud, a fully managed and autoscal-
ing DBaaS oering. While this paper focuses on the on-premise
mode, we plan to describe the architecture of ClickHouse Cloud in
a follow-up publication. The standalone mode turns ClickHouse
into a command line utility for analyzing and transforming les,
making it a SQL-based alternative to Unix tools like
cat
and
grep
.
4
While this requires no prior conguration, the standalone mode is
restricted to a single server. Recently, an in-process mode called
chDB [
15
] has been developed for interactive data analysis use cases
like Jupyter notebooks [
37
] with Pandas dataframes [
61
]. Inspired
by DuckDB [
67
], chDB embeds ClickHouse as a high-performance
OLAP engine into a host process. Compared to the other modes,
this allows to pass source and result data between the database
engine and the application eciently without copying as they run
in the same address space.
5
3 STORAGE LAYER
This section discusses MergeTree* table engines as ClickHouse’s
native storage format. We describe their on-disk representation and
discuss three data pruning techniques in ClickHouse. Afterwards,
we present merge strategies which continuously transform data
without impacting simultaneous inserts. Finally, we explain how
updates and deletes are implemented, as well as data deduplication,
data replication, and ACID compliance.
3
Blog post: clickhou.se/keeper
4
Blog posts: clickhou.se/local, clickhou.se/local-fastest-tool
5
Blog post: clickhou.se/chdb-rocket-engine
3732
文档被以下合辑收录
相关文档
评论