导读
TiDB Serverless 上的向量化功能终于开始邀约体验啦!本文是来自 TiDB 社区用户对 TiDB Vector 功能初体验的详细分享,hey-hoho 介绍了他从申请体验到实际操作的全过程,包括创建 TiDB Vector 实例、进行向量检索的初体验,以及实现以图搜图和自然语言搜图的基础应用。如果你对 TiDB Serverless 感兴趣,欢迎了解 TiDB Vector,一起开启 TiDB Serverless 数据库之旅吧!
作者丨hey-hoho
来自神州数码钛合金战队
神州数码钛合金战队是一支致力于为企业提供分布式数据库 TiDB 整体解决方案的专业技术团队。团队成员拥有丰富的数据库从业背景,全部拥有 TiDB 高级资格证书,并活跃于 TiDB 开源社区,是官方认证合作伙伴。目前已为 10+客户提供了专业的 TiDB 交付服务,涵盖金融、证券、物流、电力、政府、零售等重点行业。
最早知道 TiDB 要支持向量化的消息应该是在 23 年 10 月份左右,到第一次见到 TiDB Vector 的样子是在今年 1 月初,当时 dongxu 在朋友圈发了一张图:
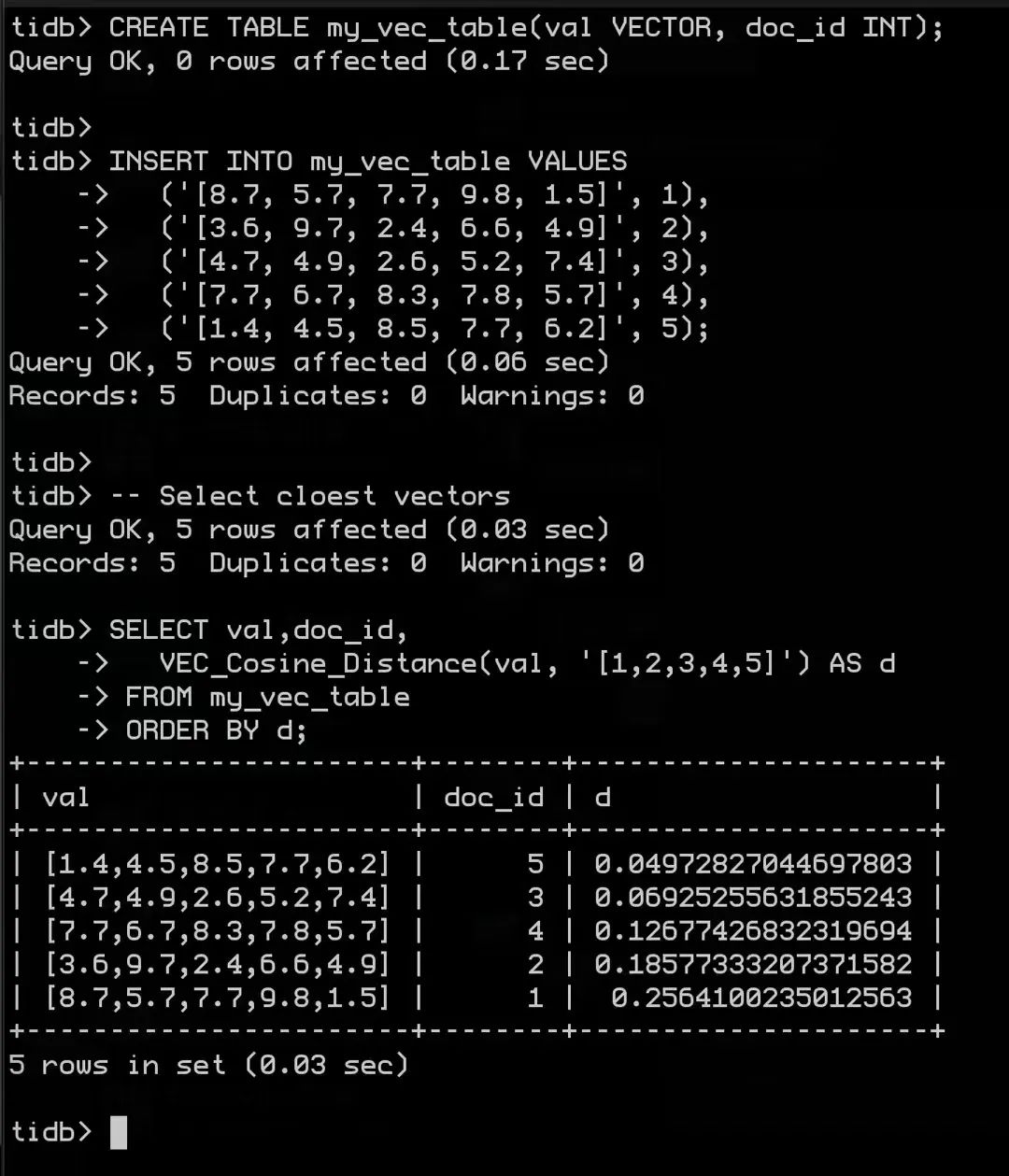
waitlist 申请入口:https://tidb.cloud/ai 体验入口:https://tidbcloud.com/
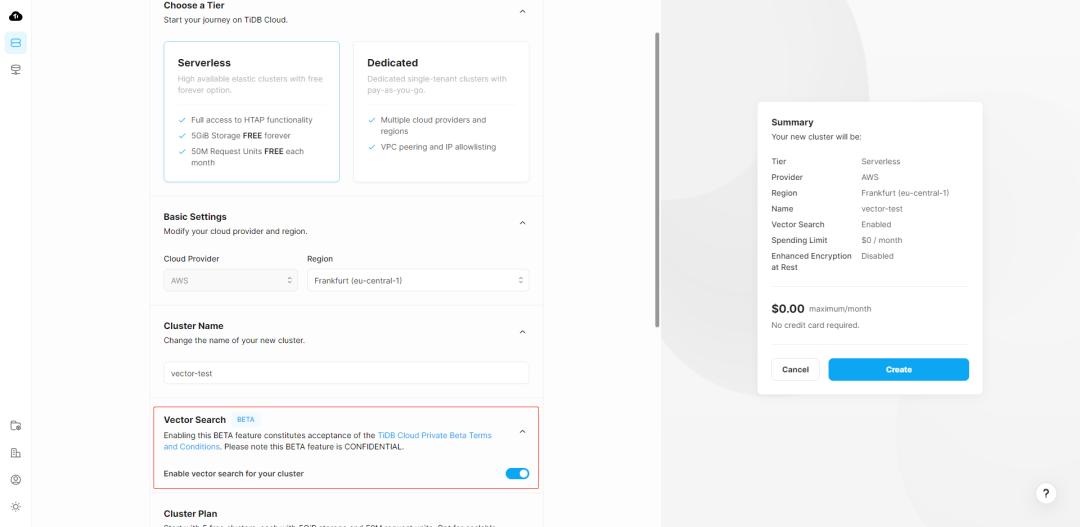
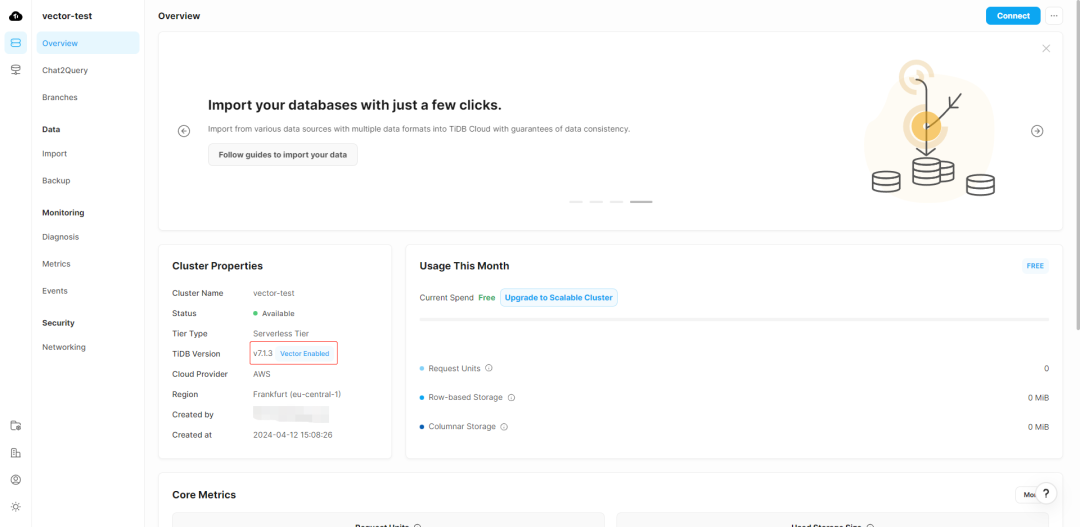
向量:向量就是一组浮点数,在编程语言中通常体现为 float 数组,数组的长度叫做维度(dim),维度越大精度越高,向量的数学表示是多维坐标系中的一个点。例如 RGB 颜色表示法就是一个简单的向量示例。
embedding:中文翻译叫嵌入,感觉不好理解,实质上就是把非结构化数据(文本、语音、图片、视频等)通过一系列算法加工变成向量的过程,这里面的算法叫做模型(model)。
向量检索:计算两个向量之间的相似度。
CREATE TABLE vector_table (
id int PRIMARY KEY,
doc TEXT,
embedding vector < float > (3)
);复制
INSERT INTO vector_table VALUES (1, 'apple', '[1,1,1]'), (2, 'banana', '[1,1,2]'), (3, 'dog', '[2,2,2]');
复制
SELECT *, vec_cosine_distance(embedding, '[1,1,3]') as distance FROM vector_table ORDER BY distance LIMIT 3;
+-----------------------+-----------------------+---------------------+
| id | doc | embedding | distance |
+-----------------------+-----------------------+---------------------+
| 2 | banana | [1,1,2] | 0.015268072165338209|
| 3 | dog | [2,2,2] | 0.1296117202215108 |
| 1 | apple | [1,1,1] | 0.1296117202215108 |
+---------+-------------+-----------------------+---------------------+复制
这里的 distance 就是两个向量之间的相似度,这个相似度是用 vec_cosine_distance 函数计算出来的,意味着两个向量之间的夹角越小相似性越高,夹角大小用余弦值来衡量。
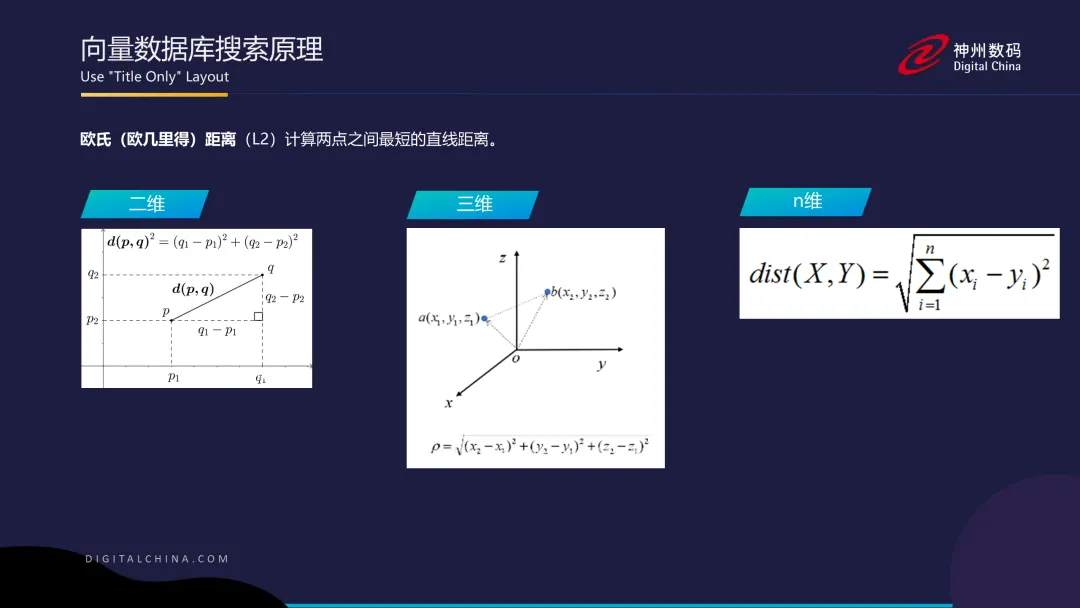
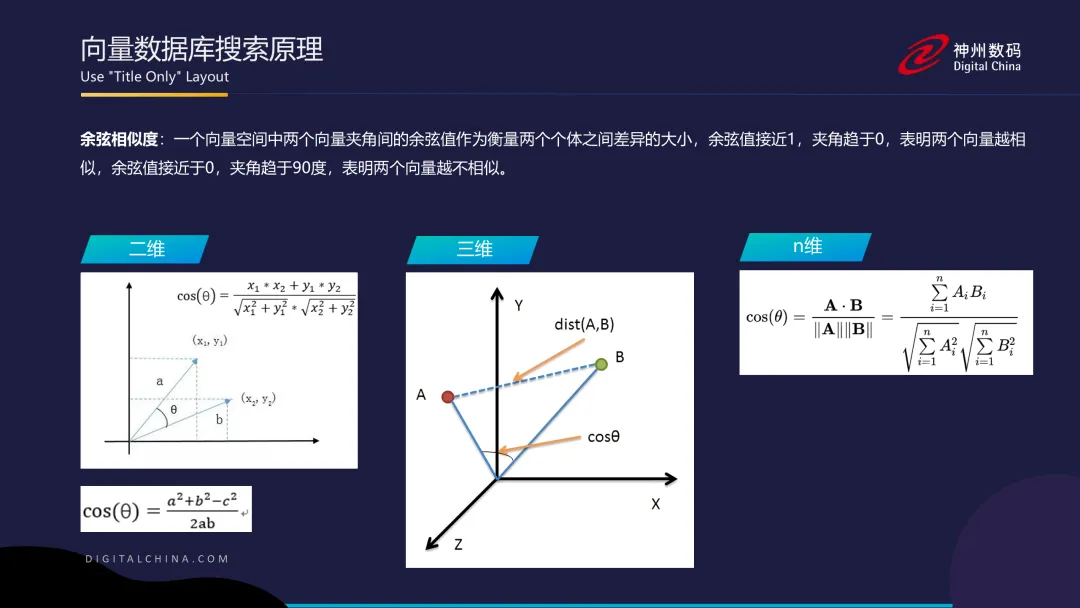

import pymysql
def GetConnection():
connection = pymysql.connect(
host = "xxx.xxx.prod.aws.tidbcloud.com",
port = 4000,
user = "xxx.root",
password = "xxx",
database = "test",
ssl_verify_cert = True,
ssl_verify_identity = True,
ssl_ca = "C:\\Users\\59131\\Downloads\\isrgrootx1.pem"
)
return connection复制
再借助 Towhee 来简化 embedding 的处理,里面包含了常用的非结构化数据到向量数据的转换模型,用流水线(pipeline)的形式清晰构建整个处理过程。
from towhee import ops,pipe,AutoPipes,AutoConfig,DataCollection
image_pipe = AutoPipes.pipeline('text_image_embedding')复制
@AutoConfig.register
class TextImageEmbeddingConfig(BaseModel):
model: Optional[str] = 'clip_vit_base_patch16'
modality: Optional[str] = 'image'
customize_embedding_op: Optional[Any] = None
normalize_vec: Optional[bool] = True
device: Optional[int] = -1复制
create table if not exists img_list
(
id int PRIMARY KEY,
path varchar(200) not null,
embedding vector<float>(512)
);复制
def LoadImage(connection):
cursor = connection.cursor()
cursor.execute("create table if not exists img_list (id int PRIMARY KEY, path varchar(200) not null, embedding vector<float>(512));")
img_dir='D:\\\\test\\\\'
files = os.listdir(img_dir)
for i in range(len(files)):
path=os.path.join(img_dir, files[i])
embedding = image_pipe(path).get()[0]
cursor.execute("INSERT INTO img_list VALUE ("+str(i)+",'"+path+"' , '"+np.array2string(embedding, separator=',')+"');")
connection.commit()复制
如果用 ORM 框架的话这里对数据库和向量加工操作会简单些,不需要数组到字符串之间的手工转换。
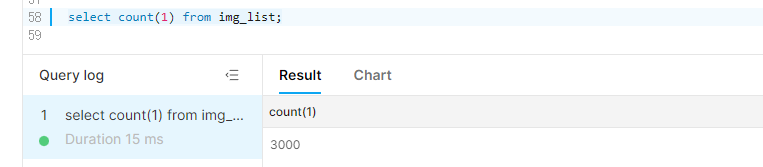
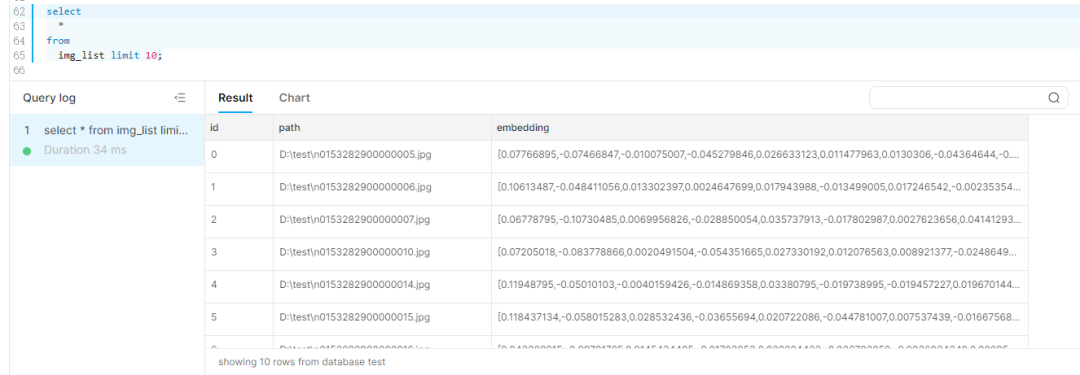
def SearchInTiDB(connection,vector):
cursor = connection.cursor()
begin_time = datetime.datetime.now()
cursor.execute("select id,path,vec_cosine_distance(embedding, '"+np.array2string(vector, separator=',')+"') as distance from img_list order by distance limit 3;")
end_time=datetime.datetime.now()
print("Search time:",(end_time-begin_time).total_seconds())
df =pd.DataFrame(cursor.fetchall())
return df[1]复制
def read_images(img_paths):
imgs = []
op = ops.image_decode.cv2_rgb()
for p in img_paths:
imgs.append(op(p))
return imgs
def ImageSearch(connection,path):
emb = image_pipe(path).get()[0]
res = SearchInTiDB(connection,emb)
p = (
pipe.input('path','search_result')
.map('path', 'img', ops.image_decode.cv2('rgb'))
.map('search_result','prev',read_images)
.output('img','prev')
)
DataCollection(p(path,res)).show()复制
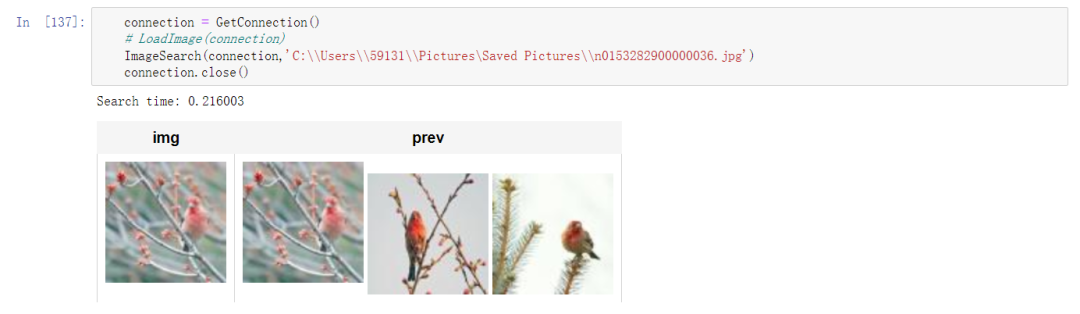
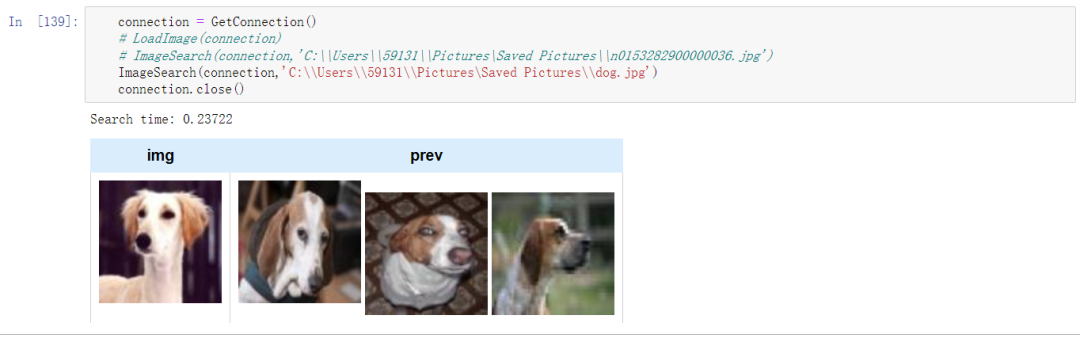
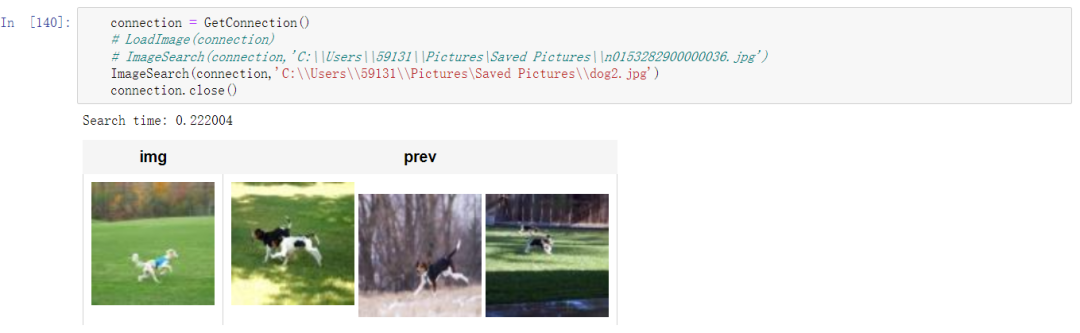
create table if not exists img_list_hnsw
(
id int PRIMARY KEY,
path varchar(200) not null,
embedding vector<float>(512) COMMENT "hnsw(distance=cosine)"
);复制
E:\GitLocal\AITester>python tidb_vec.py
Search time: 0.320241
+------------------------------------+------------------------------------------------------------------------------------------------------+
| img | prev |
+====================================+======================================================================================================+
| Image shape=(900, 900, 3) mode=RGB | [Image shape=(84, 84, 3) mode=RGB,Image shape=(84, 84, 3) mode=RGB,Image shape=(84, 84, 3) mode=RGB] |
+------------------------------------+------------------------------------------------------------------------------------------------------+
E:\GitLocal\AITester>python tidb_vec.py
Search time: 0.239746
+------------------------------------+------------------------------------------------------------------------------------------------------+
| img | prev |
+====================================+======================================================================================================+
| Image shape=(900, 900, 3) mode=RGB | [Image shape=(84, 84, 3) mode=RGB,Image shape=(84, 84, 3) mode=RGB,Image shape=(84, 84, 3) mode=RGB] |
+------------------------------------+------------------------------------------------------------------------------------------------------+复制

def TextSearch(connection,text):
text_conf = AutoConfig.load_config('text_image_embedding')
text_conf.modality = 'text'
text_pipe = AutoPipes.pipeline('text_image_embedding', text_conf)
embedding = text_pipe(text).get()[0]
res=SearchInTiDB(connection,embedding)
p = (
pipe.input('text','search_result')
.map('search_result','prev',read_images)
.output('text','prev')
)
DataCollection(p(text,res)).show()复制
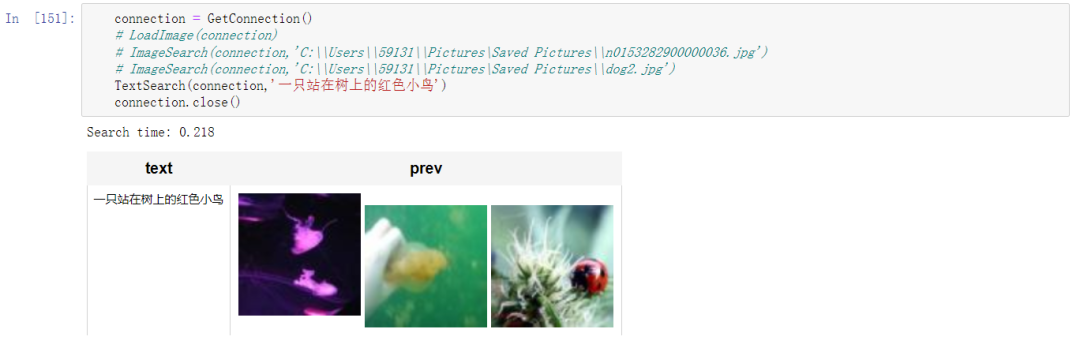
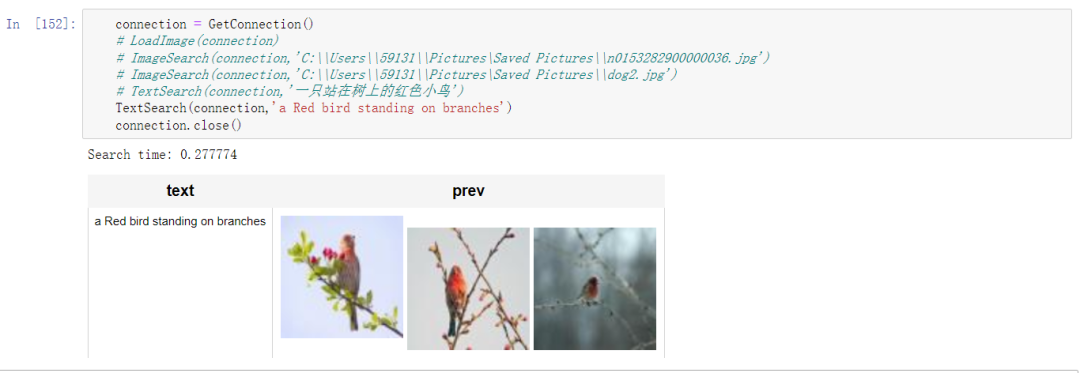
/ 相关推荐 /
AmzTrends x TiDB Serverless:通过云原生改造实现全局成本降低 80%
