01
Topic 管理
消息的生命周期:不同类型的消息可能需要不同的保留时间
2. 数据量:预估每个 Topic 的数据增长速度和总量
3. 访问模式:是读密集型还是写密集型
4. 可靠性要求:是否需要高可靠性,影响复制因子的设置
5. 性能需求:吞吐量和延迟的要求
// 代码示例
public class KafkaTopicManager {
private final AdminClient adminClient;
public KafkaTopicManager(Properties props) {
this.adminClient = AdminClient.create(props);
}
创建 Topic
public void createTopic(String topicName, int numPartitions, short replicationFactor) {
NewTopic newTopic = new NewTopic(topicName, numPartitions, replicationFactor);
可以设置 Topic 的配置
Map<String, String> configs = new HashMap<>();
configs.put("cleanup.policy", "delete"); 清理策略
configs.put("retention.ms", "604800000"); 消息保留时间,默认7天
newTopic.configs(configs);
try {
CreateTopicsResult result = adminClient.createTopics(
Collections.singleton(newTopic)
);
等待操作完成
result.all().get(10, TimeUnit.SECONDS);
System.out.println("Topic " + topicName + " created successfully");
} catch (Exception e) {
System.err.println("Failed to create topic: " + e.getMessage());
}
}
修改 Topic 配置
public void updateTopicConfig(String topicName, Map<String, String> updateConfigs) {
ConfigResource resource = new ConfigResource(
ConfigResource.Type.TOPIC, topicName
);
Collection<AlterConfigOp> configOps = updateConfigs.entrySet().stream()
.map(entry -> new AlterConfigOp(
new ConfigEntry(entry.getKey(), entry.getValue()),
AlterConfigOp.OpType.SET
))
.collect(Collectors.toList());
Map<ConfigResource, Collection<AlterConfigOp>> configs =
Collections.singletonMap(resource, configOps);
try {
adminClient.incrementalAlterConfigs(configs).all().get();
System.out.println("Topic configuration updated successfully");
} catch (Exception e) {
System.err.println("Failed to update topic config: " + e.getMessage());
}
}
}
复制
最佳实战建议
Topic 配置优化:
根据业务需求设置合适的保留时间
对于重要数据设置适当的复制因子(通常为3)
根据消息大小和频率选择合适的段文件大小
2. 运维管理:
定期监控 Topic 的状态
及时清理不再使用的 Topic
做好容量规划
3. 安全考虑:
实施适当的访问控制
配置 SSL/SASL 认证
做好备份和恢复策略
02
分区管理
分区的物理存储:
每个分区对应一个日志目录
消息按照追加写入的方式存储
通过索引文件加速消息查找
2. 分区的复制机制:
每个分区可以有多个副本
一个 Leader 副本负责读写
多个 Follower 副本负责同步数据
3. 分区的负载均衡:
分区在 Broker 间均匀分布
副本在不同 Broker 上分散存储
自动进行分区重分配
// 分区管理代码示例与解释
public class KafkaPartitionManager {
private final AdminClient adminClient;
private static final Logger logger = LoggerFactory.getLogger(KafkaPartitionManager.class);
public KafkaPartitionManager(Properties props) {
this.adminClient = AdminClient.create(props);
}
分区扩展
public void increasePartitions(String topicName, int newPartitionCount) {
首先检查当前分区数
try {
TopicDescription topicDescription = adminClient.describeTopics(
Collections.singleton(topicName)
).values().get(topicName).get();
int currentPartitions = topicDescription.partitions().size();
if (newPartitionCount <= currentPartitions) {
logger.warn("New partition count must be greater than current count: " +
currentPartitions);
return;
}
创建新分区
Map<String, NewPartitions> newPartitionsMap = new HashMap<>();
newPartitionsMap.put(topicName, NewPartitions.increaseTo(newPartitionCount));
adminClient.createPartitions(newPartitionsMap).all().get(30, TimeUnit.SECONDS);
logger.info("Successfully increased partitions from {} to {}",
currentPartitions, newPartitionCount);
} catch (Exception e) {
logger.error("Failed to increase partitions: " + e.getMessage(), e);
}
}
分区状态监控
public void monitorPartitionHealth(String topicName) {
try {
TopicDescription desc = adminClient.describeTopics(
Collections.singleton(topicName)
).values().get(topicName).get();
for (TopicPartitionInfo partition : desc.partitions()) {
检查副本同步状态
boolean isUnderReplicated = partition.replicas().size() > partition.isr().size();
检查 Leader 状态
boolean hasLeader = partition.leader() != null;
检查副本分布
boolean hasGoodReplicaSpread = checkReplicaSpread(partition.replicas());
记录健康状态
logger.info("Partition {} status:", partition.partition());
logger.info(" - Under replicated: {}", isUnderReplicated);
logger.info(" - Has leader: {}", hasLeader);
logger.info(" - Good replica spread: {}", hasGoodReplicaSpread);
/ 如果发现问题,发出警告
if (isUnderReplicated || !hasLeader || !hasGoodReplicaSpread) {
alertPartitionIssue(topicName, partition.partition());
}
}
} catch (Exception e) {
logger.error("Failed to monitor partition health: " + e.getMessage(), e);
}
}
// 检查副本分布是否合理
private boolean checkReplicaSpread(List<Node> replicas) {
Set<Integer> brokerIds = replicas.stream()
.map(Node::id)
.collect(Collectors.toSet());
// 检查副本是否分布在不同的 Broker 上
return brokerIds.size() == replicas.size();
}
// 分区重平衡
public void rebalancePartitions(String topicName) {
try {
// 获取当前分区分配情况
Map<Integer, List<TopicPartitionInfo>> brokerToPartitions = new HashMap<>();
TopicDescription desc = adminClient.describeTopics(
Collections.singleton(topicName)
).values().get(topicName).get();
// 分析分区分布
for (TopicPartitionInfo partition : desc.partitions()) {
int leaderId = partition.leader().id();
brokerToPartitions.computeIfAbsent(leaderId, k -> new ArrayList<>())
.add(partition);
}
// 检查是否需要重平衡
if (needsRebalancing(brokerToPartitions)) {
// 创建重平衡计划
Map<TopicPartition, Optional<NewPartitionReassignment>> reassignments =
createReassignmentPlan(brokerToPartitions);
// 执行重平衡
adminClient.alterPartitionReassignments(reassignments).all().get();
logger.info("Partition rebalancing initiated for topic {}", topicName);
}
} catch (Exception e) {
logger.error("Failed to rebalance partitions: " + e.getMessage(), e);
}
}
}
复制
分区管理最佳建议
分区扩展原则:
只能增加分区数量,不能减少
增加分区会影响消息的顺序性
建议在低峰期进行分区扩展
2. 监控和维护:
定期检查分区的健康状态
监控分区的负载均衡情况
及时处理副本同步问题
3. 性能优化:
合理设置分区大小
优化分区的分布
定期进行日志压缩
03
如何选择合适的分区数
1. 影响分区数量选择的关键因素:
吞吐量需求
单个分区的吞吐量上限
生产者和消费者的并发度
消息大小和频率
2. 硬件资源
服务器CPU核心数
可用内存大小
磁盘I/O能力
网络带宽
消息顺序性要求
延迟敏感度
数据可靠性要求
public class PartitionCalculator {
private static final Logger logger = LoggerFactory.getLogger(PartitionCalculator.class);
// 计算建议的分区数
public PartitionRecommendation calculateOptimalPartitions(
KafkaClusterMetrics clusterMetrics,
BusinessRequirements requirements) {
// 基于吞吐量的计算
int throughputBasedCount = calculateThroughputBasedPartitions(
requirements.getTargetThroughput(),
clusterMetrics.getPerPartitionThroughput()
);
// 基于消费者的计算
int consumerBasedCount = calculateConsumerBasedPartitions(
requirements.getConsumerCount(),
requirements.getConcurrencyFactor()
);
// 基于资源的限制
int resourceLimitedCount = calculateResourceLimitedPartitions(
clusterMetrics.getAvailableMemory(),
clusterMetrics.getCpuCores(),
clusterMetrics.getDiskIOCapacity()
);
// 综合考虑各种因素
int recommendedCount = Math.min(
Math.max(throughputBasedCount, consumerBasedCount),
resourceLimitedCount
);
return new PartitionRecommendation(
recommendedCount,
generateRecommendationReport(
throughputBasedCount,
consumerBasedCount,
resourceLimitedCount,
recommendedCount
)
);
}
// 分区性能监控
public class PartitionPerformanceMonitor {
private final KafkaConsumer<?, ?> consumer;
private final Map<TopicPartition, PartitionMetrics> metricsMap = new ConcurrentHashMap<>();
public void startMonitoring(String topicName) {
ScheduledExecutorService executor = Executors.newSingleThreadScheduledExecutor();
executor.scheduleAtFixedRate(() -> {
try {
// 收集分区性能指标
collectPartitionMetrics(topicName);
// 分析性能数据
analyzePartitionPerformance();
// 生成建议
generateOptimizationSuggestions();
} catch (Exception e) {
logger.error("Error monitoring partitions: " + e.getMessage(), e);
}
}, 0, 1, TimeUnit.MINUTES);
}
private void collectPartitionMetrics(String topicName) {
consumer.assignment().forEach(partition -> {
if (partition.topic().equals(topicName)) {
// 收集延迟指标
long latency = measurePartitionLatency(partition);
// 收集吞吐量指标
double throughput = measurePartitionThroughput(partition);
// 更新指标
metricsMap.compute(partition, (k, v) -> {
if (v == null) {
return new PartitionMetrics(latency, throughput);
}
return v.update(latency, throughput);
});
}
});
}
private void analyzePartitionPerformance() {
metricsMap.forEach((partition, metrics) -> {
// 检查性能问题
if (metrics.getAverageLatency() > 100) { // 延迟阈值
logger.warn("High latency detected in partition {}: {} ms",
partition.partition(), metrics.getAverageLatency());
}
if (metrics.getAverageThroughput() < 1000) { // 吞吐量阈值
logger.warn("Low throughput detected in partition {}: {} msgs/sec",
partition.partition(), metrics.getAverageThroughput());
}
});
}
}
}
复制
分区数量选择的具体建议
初始分区数量设置:
建议从较小的数量开始(如分区数 = 2 Broker数)
预留30%的增长空间
考虑未来扩展需求
2. 性能监控指标:
分区延迟:通常应保持在100ms以下
分区吞吐量:根据业务需求设定基准线
资源使用率:CPU、内存、磁盘I/O等
3. 调整时机:
当观察到性能瓶颈时
集群扩容时
业务需求发生重大变化时
4. 注意事项:
增加分区数是不可逆的操作
分区数增加会影响消息顺序
需要考虑分区再平衡的开销
我们可以看到对 Kafka Topic 和分区管理机制在分布式消息系统中扮演着至关重要的角色。合理的 Topic 设计能够更好地组织和管理消息流,而优化的分区配置则能够充分发挥集群的性能潜力。在实际应用中,需要根据具体的业务场景、性能需求和硬件资源来权衡各种因素,选择最适合的配置方案。同时,持续的监控和优化也是确保系统稳定运行的关键。
04
加群请添加作者
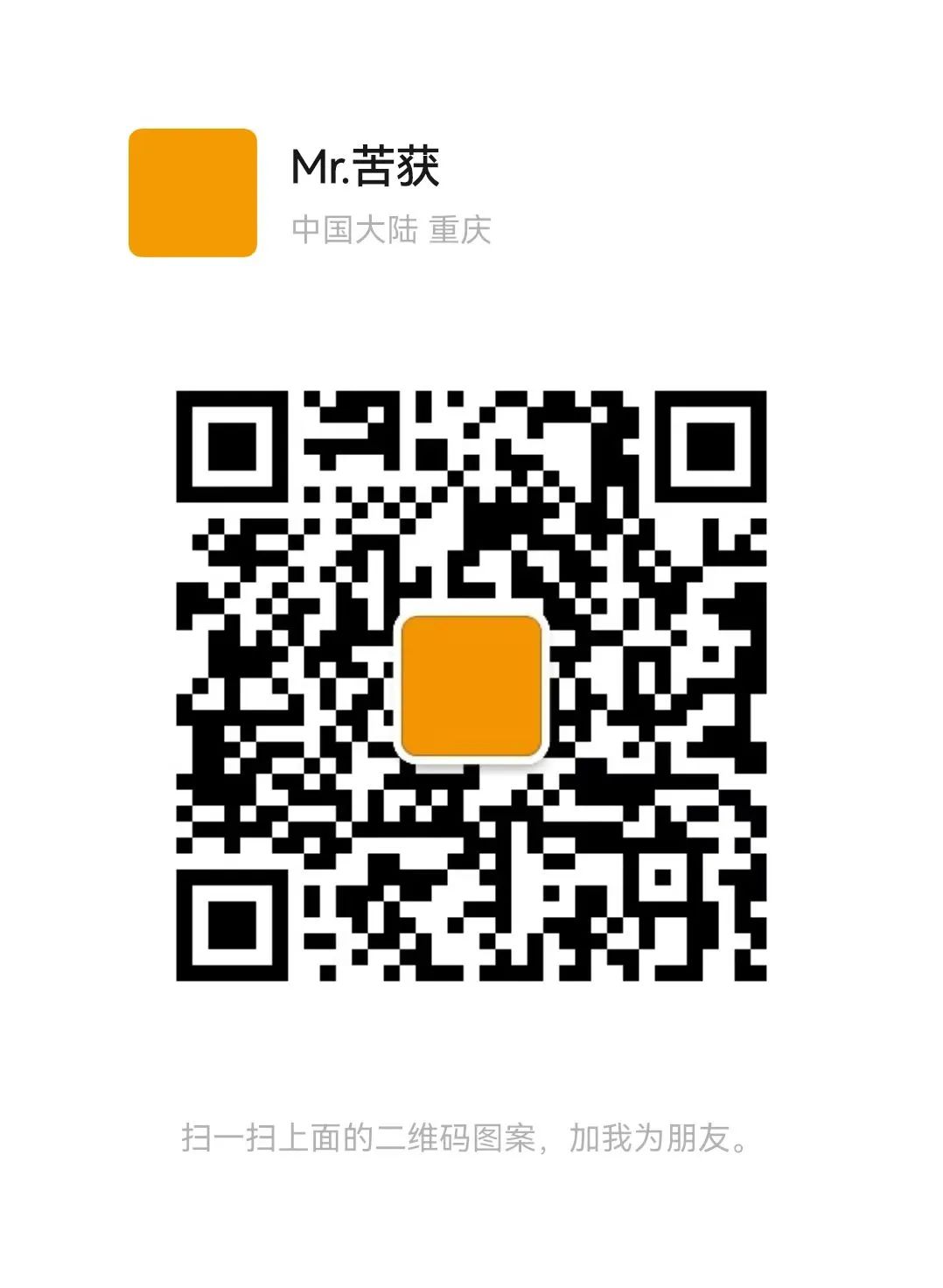
05
获取文档及视频资料
推荐阅读系列文章
建议收藏 | Dinky系列总结篇 建议收藏 | Flink系列总结篇 建议收藏 | Flink CDC 系列总结篇 建议收藏 | Doris实战文章合集 建议收藏 | Seatunnel 实战文章系列合集 建议收藏 | 实时离线输数仓(数据湖)总结篇 建议收藏 | 实时离线数仓实战第一阶段总结
如果喜欢 请点个在看分享给身边的朋友