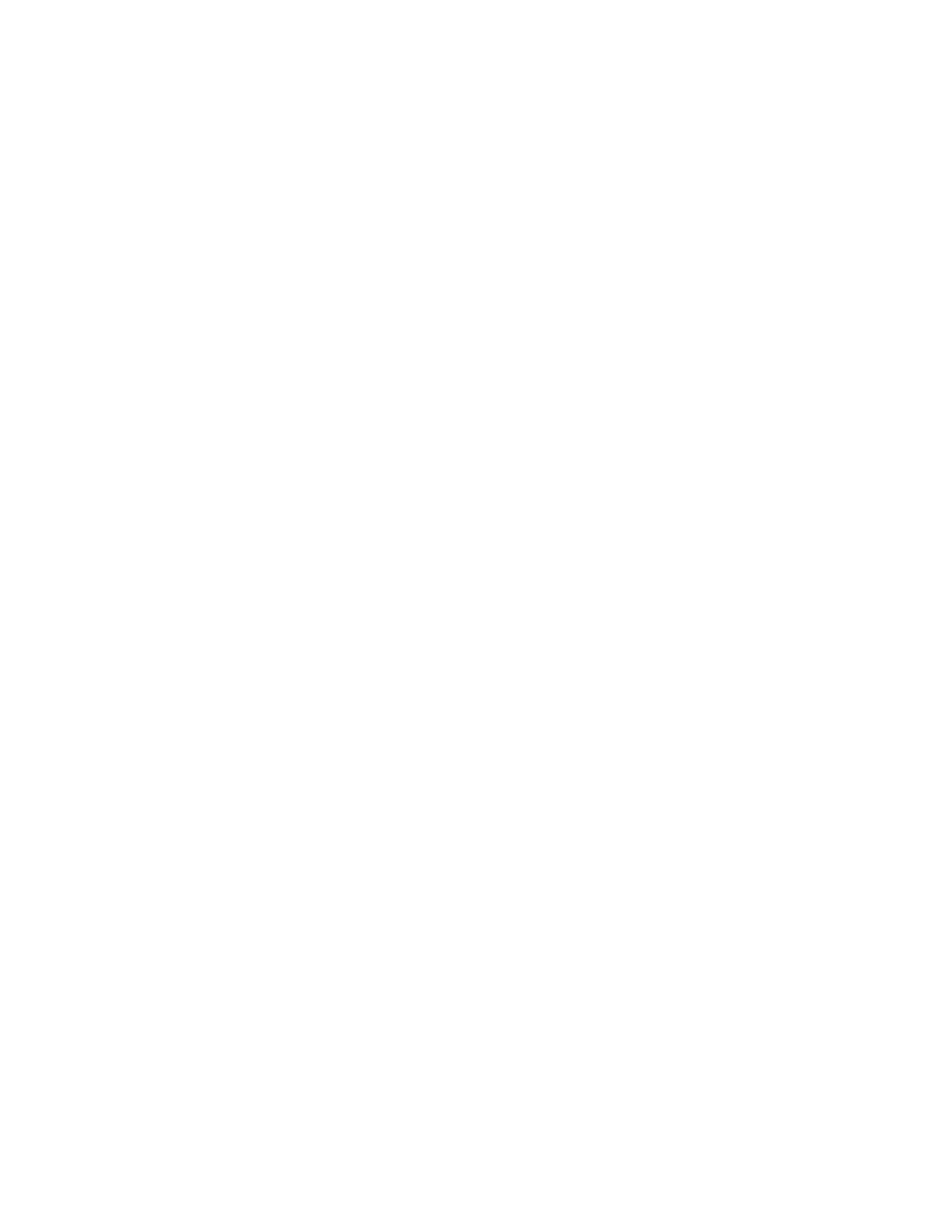
ADF2T: an Active Disk Failure Forecasting and Tolerance Software
Hongzhang Yang
†‡
, Yahui Yang
ѽ
†
, Zhengguang Chen
‡
, Zongzhao Li
‡
and Yaofeng Tu
ѽ
‡§
†
School of Software & Microelectronics, Peking University, China
‡
ZTE Corporation, China
§
State Key Laboratory of Mobile Network and Mobile Multimedia Technology, China
*Corresponding author: Yahui Yang(yhyang@ss.pku.edu.cn), Yaofeng Tu(tu.yaofeng@zte.com.cn)
Abstract—The reliability of distributed file system is
inevitably affected by hard disk failure. This paper proposes an
active disk failure forecasting and tolerance software. Firstly,
multiple SMART records in the time window are merged into
one sample, and after sliding, tens of times of positive samples
are created.
, the features are selected by two-stage
sorting method, so that the most conducive features are used in
machine learning modeling, and the time for model training can
be shortened obviously. Thirdly, through two-stage verification,
parameters can be adjusted in time for unreasonable proactive
reconstruction strategies. Experiments show that modeling and
forecast of ZTE data set and Backblaze data set respectively, the
recall rate is 95.66% and 84.28%, and the error rate is 0.23%
and 2.45%. The work in this paper has been commercially used
for more than one year in ZTE data center. The reliability of
distributed file system software is significantly improved.
Keywords—reliability; disk; failure; forecast
I. INTRODUCTION
As a typical large-scale software system, the reliability of
distributed file system is inevitably affected by hardware
failure, especially hard disk failure. According to the IDC
white paper [1], the data all over the world will reach 175ZB
by the year of 2025. Currently, the annual failure rate of disks
is about 1% [2]. So, hundreds of millions of failure disks will
appear each year worldwide soon. It is undeniable that disk
failure has become the main failure source in data center [3].
According to the survey results provided by ZTE Corporation,
among all the failures in the ZTE data center in 2019, disk
failures accounted for more than half, namely 53.49%.
Similarly, in other research works, this number was 71.1% [4]
and 49.1% [2]. Disk failure can directly lead to disastrous
consequences, such as data loss and business interruption.
In the traditional distributed file systems, it is data
redundancy that mainly ensures reliability, including replica,
erasure code, snapshot, and so on. After disks failed, these
technologies rely on redundancy to ensure read-write
operations and data recovery, so all of them are passive
processing technologies. The defects are as follows: 1) when
the number of failed disks is more than the number of
redundant disks, the risk of permanent data loss is
significantly; 2) in the process of data recovery, system
resources are inevitably occupied by data recovery; 3)
redundancy takes up extra storage space, which leads to the
increase of cos
t. Different from above technologies, this
paper proposes an active disk fault-tolerance technology, that
is, by forecasting disk failure, it provides possibility to
migrate data in advance, as a result the reliability of the
distributed file system is ensured.
A microcosmic view to disk failure, is just as human
beings experience “healthy, sub-healthy, illness, and death”
in their own lifetimes, disk individuals also experience
"healthy, sub-healthy, near-failure, and failure" inevitably.
This is because of the wear of various hardware components.
At the same time, similar to human death due to congenital
physical defects, and accidental deaths caused by accidents
such as car accidents, earthquakes, disasters, animal attacks,
etc. Disks also have unforecastable failures caused by
manufacturing defects or accidental failures, such as
formaldehyde, vibration, sudden voltage changes, excessive
air humidity, and so on. Obviously, the inevitable failure of
the disk has a near-term failure window period, which has the
possibility of forecast, but the accidental failure of the disk is
often sudden, and there is absolutely no near-failure window
period, so it is not forecastable. Considering ZTE's
experience in datacenter operation, this paper gives the
definition of each state of disk as:
x The healthy state, is that the disk can correctly
perform operations such as mount, read, write, and the
operation response time meets the specifications of
the disk manufacturer's manual, and the disk SMART
(Self-Monitoring Analysis and Reporting Technology)
[5] result is normal.
x The sub-healthy state, is that the disk occasionally
freezes, and the response time of operations such as
mount, read, and write does not meet the
specifications of the disk manufacturer's manual
occasionally, and the SMART result of the disk are
slightly abnormal.
x The near-failure state, is one or more of the following
conditions on the disk: mechanical noise, severe
abnormality of the SMART result of the disk, data
loss, read or write incompletely, read or write slowly,
frequent offline, and high temperature, among which
read or write slowly is the most common. That is, the
waiting time of read or write requests in the queue for
20 consecutive times exceeds 1.5 seconds.
x The failure state, is one or more of the following
conditions: file system log printing Medium Error, IO
Error, critical target error, Metadata IO Error, mount
failed, no response to read or write, etc.
The disk failure forecast technology is to find the trends
that the disk is on the near-failure state, thereby forecasting
that the disk will become failure state in the foreseeable
future, for example 7 days.
13
2020 IEEE International Symposium on Software Reliability Engineering Workshops (ISSREW)
978-1-7281-7735-9/20/$31.00 ©2020 IEEE
DOI 10.1109/ISSREW51248.2020.00030
Authorized licensed use limited to: ZTE CORPORATION. Downloaded on July 26,2023 at 12:22:01 UTC from IEEE Xplore. Restrictions apply.
相关文档
评论