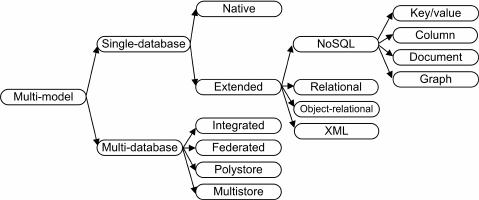
in big data analytics [40], or big time-series data manage-
ment [35], i.e., different aspects of big data challenges.
2. COVERED TOPICS
2.1 Background, History and Classification
In the first part of the tutorial we first provide a mo-
tivating example of a multi-model application and briefly
describe most common data models used in the world of
multi-model DBMSs (mainly key/value, relational, JSON,
XML, and graph). Next, we focus on their history and clas-
sification.
The world of multi-model DBMSs can be divided into
single-database and multi-database (see Figure 1), depend-
ing on whether the multiple models are handled in a single
DBMS or there exist a number of cooperating or centrally
managed DBMSs, each handling own data model(s).
Figure 1: Classification of multi-model data man-
agement systems
The first approaches towards multi-model multi-database
data management can be seen in integrated DBMSs [37] and
federated DBMSs [20, 36]. Both types of systems can be
characterized as a meta-DBMS consisting of a collection of
(possibly) heterogeneous DBMSs which can differ in data
models, constraints, query languages, and/or transaction
management. The data integration is usually based on the
idea of mediators [43]. The main difference is that in fed-
erated systems the DBMSs are autonomous and cooperate.
Thus federated databases provide a compromise between no
integration (where the users must explicitly interface with
multiple autonomous DBMSs) and total integration (where
the users can access data through a single global interface
but cannot directly access a DBMS as a local user) [36].
Recently there has appeared a successor of federated data-
bases – so-called polystore systems [38]. The key represen-
tative, system BigDAWG [17], also enables users to pose
declarative queries that span several DBMSs. However, it
consists of islands of information, i.e. collections of DBMSs
accessed with a single query language (e.g., relational or ar-
ray). Cross-island queries are supported using casting (e.g.,
tables to arrays or vice versa).
Another recent related approach from the area of big data
analytics represent so-called multistore systems [23, 44]. For
example system MISO [23] involves two types of data stores
– a parallel relational data warehouse and a system for mas-
sive data storage and analysis (namely HDFS with Apache
Hive). The aim is to combine their capabilities in order to
gain more efficient query processing.
Multi-model single-database DBMSs can also be further
classified. Probably the most natural classification is ac-
cording their origin [2] (see Figure 1). Similarly to XML
databases, we can distinguish native and extended DBMSs
depending on whether the support for multiple models was
the initial feature of the system, or it was added later. In
the latter case we can find representatives amongst all four
core types of NoSQL databases as well as traditional DBMS.
2.2 Overview and Comparison
In the second part of the tutorial we take a closer look
at particular multi-model single-database DBMSs from the
point of view of three key aspects of a database system.
The first database challenge is to develop a strategy to
store distinct data models. Approaches used in the ex-
isting multi-model DBMSs can be classified according to
the combination of used models. The main group (systems
such as, e.g., PostgreSQL or Microsoft SQL Server [9]) is
naturally represented by the (object-)relational model ex-
tended towards other data models, such as JSON, XML etc.
From the set of NoSQL databases we can observe the ten-
dency towards multi-model data management among col-
umn stores [4], key/value stores [11], or graph databases [7].
And there are also representatives of native hierarchical data
stores [5] which support other types of data models.
The second database challenge is a query language capa-
ble of accessing and combining data having distinct models.
Naturally, having a single language for managing queries
over both (semi-)structured and NoSQL data is convenient
to users. And again, in general, this is not a new fea-
ture of a query language, as we can see, e.g., in the case
of the SQL/XML [21] extension of SQL. Most of the cur-
rent NoSQL multi-model databases across the spectrum of
storage strategies [6, 4, 7] support an SQL-like language.
However, as we will show, despite this approach is natural
and user-friendly, there are significant differences as well as
persisting limitations. There also exist XML or JSON query
language extensions towards other data models (e.g., Mark-
Logic’s XPath for JSON [3]), as well as specific languages
like, e.g., SQL++[31], JSONiq [33], or FSD domain-specific
language [24]. In a more broader scope paper [32] identifies
a subset of SQL for access to NoSQL systems or paper [13]
evaluates the possibilities of using declarative structures in
NoSQL data processing. We also discuss other techniques,
like, e.g., [14, 32, 41].
The third challenge corresponds to query evaluation and
optimization. As expected, the world of multi-model DBMSs
exploits and extends verified database approaches such as in-
dices (B+ tree, inverted, range, spatial, full text, etc.), views
and materialization, hashing etc. In this part of the tutorial
we overview and compare the query optimization technolo-
gies used in the previously discussed systems. We also intro-
duce the related area of benchmarking multi-model database
systems. As more and more platforms are proposed to deal
with multi-model data, it becomes important to have bench-
marks specific for this next generation of database systems.
We mention several systems for benchmarking big data sys-
tems including YCSB [15], TPCx-BB [19], Bigframe [22],
and UniBench [25].
We conclude this part with comparison of features of the
state-of-the-art systems in the form of system-feature ma-
trices and a timeline demonstrating their evolution.
2.3 Open Problems and Challenges
In the last part of the tutorial we focus on open problems
相关文档
评论