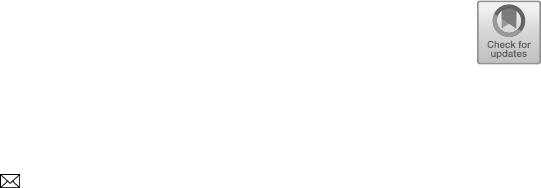
Acceleration-Guided Diffusion Model for
Multivariate Time Series Imputation
Xinyu Yang
1
, Yu Sun
1
, Shaoxu Song
2
, Xiaojie Yuan
1
, and Xinyang Chen
3
sxsong@tsinghua.edu.cn
3
School of Computer Science and Technology, Harbin Institute of Technology,
Shenzhen, China
chenxinyang@hit.edu.cn
Abstract. Multivariate time series data are pervasive in various do-
mains, often plagued by missing values due to diverse reasons. Diffusion
models have demonstrated their prowess for imputing missing values
in time series by leveraging stochastic processes. Nonetheless, a persis-
tent challenge surfaces when diffusion models encounter the task of ac-
curately modeling time series data with quick changes. In response to
this challenge, we present the Acceleration-guided Diffusion model for
Multivariate time series Imputation (ADMI). Time-series representa-
tion learning is first effectively conducted through an acceleration-guided
masked modeling framework. Subsequently, representations with a spe-
cial care of quick changes are incorporated as guiding elements in the
diffusion model, utilizing the cross-attention mechanism. Thus our model
can self-adaptively adjust the weights associated with the representation
during the denoising process. Our experiments, conducted on real-world
datasets featuring genuine missing values, conclusively demonstrate the
superior performance of our ADMI model. It excels in both imputation
accuracy and the overall enhancement of downstream applications.
Keywords: Multivariate time series · Data imputation · Self-supervised
learning · Diffusion model.
1 Introduction
Incomplete time series data are common in various fields such as meteorology
[13], traffic [6], and medical treatment [24], impairing the performance of down-
stream analysis [11]. Researchers have developed various methods for imputing
missing values in multivariate time series, based on statistics [23], machine learn-
ing [6, 15], deep learning [22, 14], etc. Because of the powerful data generation
ability, generative models [17, 28] are widely used in imputing missing values.
As one of the most advanced generative models, the diffusion model has
demonstrated impressive results in imputing time series data [28, 1, 14], tak-
ing advantages of the multi-step noise-adding and denoising processes to model
© The Author(s), under exclusive license to Springer Nature Singapore Pte Ltd. 2025
M. Onizuka et al. (Eds.): DASFAA 2024, LNCS 14851, pp. 130, 2025.
https://doi.org/10.1007/978-981-97-5779-4_8
115–
1
College of Computer Science, DISSec, Nankai University, Tianjin, China
2
Tsinghua University, Beijing, China
yangxinyu@dbis.nankai.edu.cn, {sunyu,yuanxj}@nankai.edu.cn
相关文档
评论