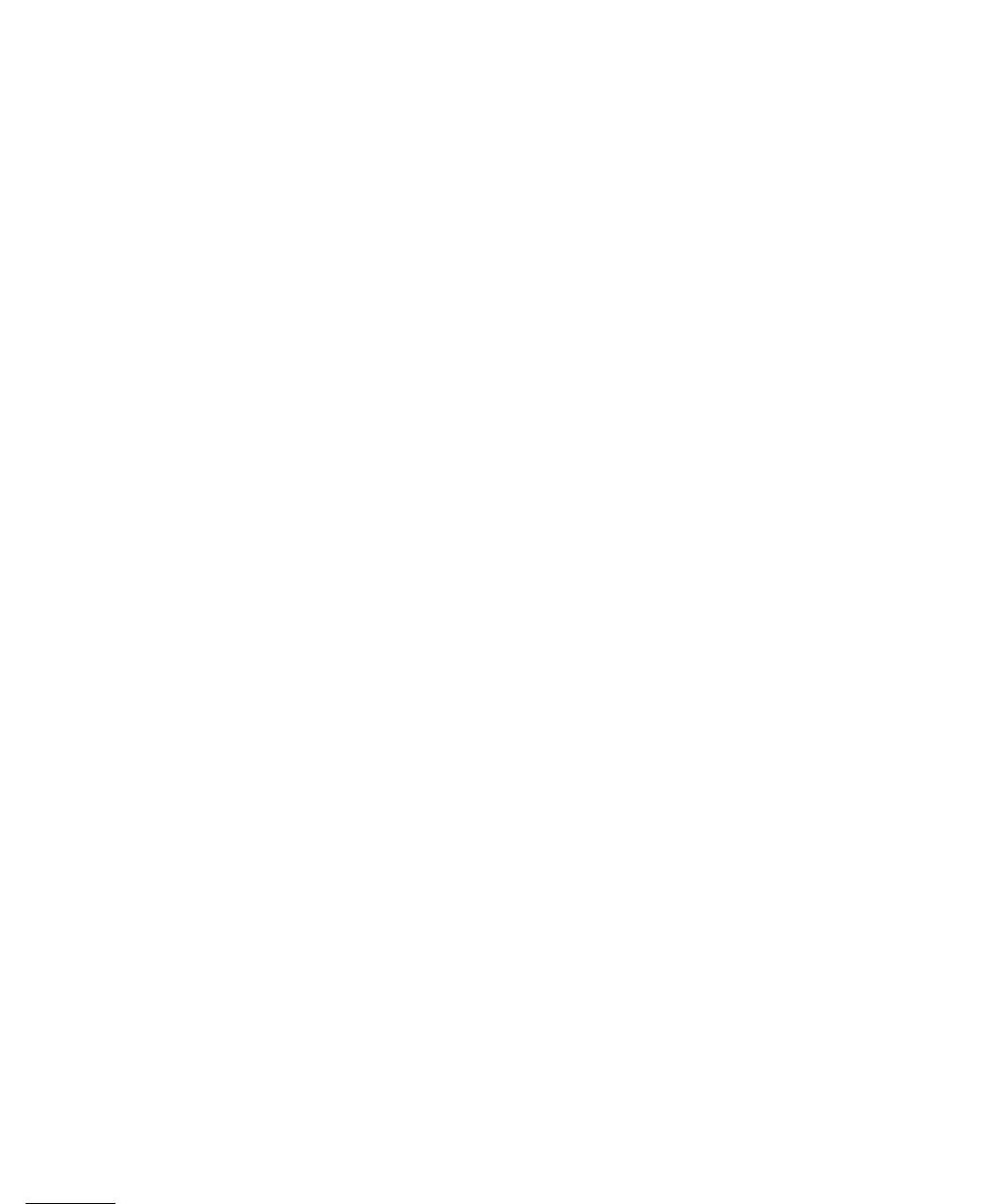
SIGMOD ’22, June 12–17, 2022, Philadelphia, PA, USA Y. Ma, S. Xie, H. Zhong, L. Lee, K.Lv
compute and storage nodes) is much higher than that of intra-
layer networking (from one compute node to another, or from one
storage node to another). Although throughput can remain ele-
vated with techniques such as pipelined and group commit [22]
adopted by existing cloud-native systems [13, 46], this largely de-
feats the goal of achieving low-latency transactions, a main pur-
pose of using a memory-optimized database engine. In terms of
reliability and availability, the direct deployment approach would
again waste much space by storing redundant copies as the stor-
age service already replicates data, similar to the cases that were
made against deploying directly a traditional database system [2,
5, 13, 46] in the cloud. Finally, the directly deployment approach
is also ill-suited in today’s cloud which is becoming more hetero-
geneous as non-x86 (notably ARM
1
) processors make inroads into
data centers. Most memory-optimized database systems were de-
signed around the x86 architecture to leverages its fast inter-core
communication and total store ordering. It was unclear how well
or whether they would work in ARM-based environments. ese
challenges and issues call for a new design that retains main-memory
performance, yet fully adapts to the cloud architecture.
We present HiEngine, Huawei’s cloud-native, memory-optimized
in-memory database engine built from scratch to tackle these chal-
lenges. A key design in HiEngine is (re)introducing persistent states
to the compute layer, to enable fast persistence and low-latency
transactions directly in the compute layer without going through
the inter-layer network on the critical path. Instead, transactions
are considered commied as soon as their log records are persisted
and replicated in the compute layer. HiEngine uses a highly paral-
lel design to store log in persistent memory on the compute side.
e log is batched and ushed periodically to the storage layer in
background to achieve high availability and reliability. is is en-
abled by SRSS [13], Huawei’s unied, shared reliable storage ser-
vice that replicates data transparently with strong consistency. In
the compute layer, SRSS leverages persistent memory such as In-
tel Optane DCPMM [12] or baery-backed DRAM [1, 47] to store
and replicate the log tail in three compute nodes. e replication
is also done in the storage layer by SRSS, but in the background
without aecting transaction latency. is way, HiEngine retains
main-memory performance in the cloud, while continuing to lever-
age what reliable storage services oers in terms of reliability and
high availability.
HiEngine also uniquely optimizes for ARM-based manycore pro-
cessors, which have been making inroads to cloud data centers as a
cost-eective and energy-ecient option. e main dierentiating
feature is that these ARM processors oer even higher core counts
their popular x86 counterparts and exhibit worse NUMA eect
in multi-socket seings. HiEngine proposes a few optimizations
to improve transaction throughput for ARM-based manycore pro-
cessors, in particular timestamp allocation and memory manage-
ment. Although there has been numerous work on main-memory
database engines that leverage modern hardware, most of them
are piecewise solutions and/or focused on particular components
(e.g., concurrency control or garbage collection). In this paper, by
1
For example, both Huawei Cloud [19] and Amazon Web Services [3] have deployed
ARM processors in their cloud oerings.
building HiEngine we share our experience and highlight the im-
portant design decisions that were rarely discussed in the research
literature for practitioners to bring and integrate ideas together to
a realize a system that is production-ready in a cloud environment.
It is also desirable to maintain backward compatibility with storage-
centric engines. Traditional cloud-native systems are gaining mo-
mentum in the market, with established ecosystem based on previ-
ous on-premise oerings. For example, Aurora, GaussDB(for MySQL)
and PolarDB all oer MySQL-compatible interfaces to allow ap-
plications to migrate easily to the cloud; Microso Socrates re-
tains the familiar SQL Server T-SQL interfaces for applications. It
is unlikely that all applications and workloads would migrate to
main-memory engines: some may not even need the high perfor-
mance provided by these engines. Similar to the adoption of main-
memory engines in on-premise environments, we believe that the
new main-memory engine needs to co-exist with existing disk-
based engines in the existing ecosystem. In later sections, we de-
scribe our approach for two database engines (HiEngine and Inn-
oDB) to co-exist in GaussDB(for MySQL) [13], Huawei’s cloud-
native, MySQL-compatible database systems oering in produc-
tion.
Finally, HiEngine introduces a few new techniques in core data-
base engine design to bridge the gap between academic prototypes
and real products in the cloud. HiEngine adopts the concept of in-
direction arrays [24] that ease data access processes, but were lim-
ited by issues such as xed static array sizes. We extend this idea
by partitioned indirection arrays to support dynamically growing
and shrinking indirection arrays, while maintaining the benets
brought by them. HiEngine also adds support for other notable fea-
tures such as index persistence and partial memory support, both
of which were oen ignored by prior research prototypes.
Next, we begin in Section 2 with recent hardware trends in mod-
ern cloud infrastructure and Huawei SRSS, which is a critical build-
ing block that enables the making of HiEngine. Section 3 rst gives
an overview of HiEngine architecture and design principles. We
then describe the detailed design of HiEngine in Sections 4–5, fol-
lowed by evaluation results in Section 6. We cover related work in
Section 7 and conclude in Section 8.
2 MODERN CLOUD INFRASTRUCTURE
is section gives an overview of recent cloud infrastructure (hard-
ware adoption and architecture) trends at Huawei Cloud which
HiEngine is built around.
2.1 Hardware Trends and Challenges
Modern cloud computing infrastructure decouples compute and
storage elements to achieve high scalability: Conceptually, one set
of nodes are dedicated to compute-intensive tasks (“compute” nodes
or instances), and another set of nodes are dedicated to storage
(“storage” nodes or instances). Most servers in cloud data centers
are x86-based and soware is thus designed around the x86 archi-
tecture which features stronger but relatively fewer cores per chip
(e.g., a couple of dozens).
Represented by products such as Amazon Graviton [3], Ampere
Altra [4] and Huawei Kunpeng [19], ARM-based manycore proces-
sors are making inroads into cloud data centers for beer price
SIGMOD ’22, June 12–17, 2022, Philadelphia, PA, USA
相关文档
评论