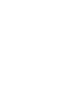
Rule-based Tuning. MySQLTuner
2
and PGTune
3
harness
database commands to gather pertinent information for tuning,
then adjust corresponding knobs according to predefined rules.
This approach, however, often restricts tuning to specific
subsets of knobs. For example, MySQLTuner and PGTune are
limited to tuning only 12 and 14 out of hundreds of MySQL
and PostgreSQL knobs, respectively.
Search-based Tuning. BestConfig [2] is a heuristic tuning
method designed to tune database knobs. It is executed in three
key steps. First, the Latin Hypercube Sampling (LHS) method
[13] is employed to sample within the knob space. Next, the
surrounding region of the best-performing sample is identified
as the new sampling space, where the LHS is conducted again.
Finally, if a sample within this new space shows improved
performance, the process returns to the second step; if not, it
reverts to the first step. What sets BestConfig apart is its highly
randomized sampling process, which may result in significant
variations in the final outcomes for the same scenario.
Gaussian Process-based Tuning. iTuned [4] pioneered Gaus-
sian process-based modeling the relationship between knobs
and database performance. By leveraging the sampling data
gathered through the LHS method, it constructs a preliminary
tuning model and employs the expected improvement method
to balance exploration and exploitation. Conversely, OtterTune
[3] builds an initial Gaussian model using historical tuning
data, and applies Lasso to identify key knobs. It then utilizes an
incremental approach [14] to dynamically increase the number
of tuning knobs throughout the process. ResTune [8] also
employs a Gaussian process, but aims to minimize system
resource utilization while maintaining DBMS performance.
Taking into account the influence of various factors such
as operating system and Java virtual machine on database
performance, CGPTuner [15] employs a Contextual Gaussian
Process Bandit Optimization to tune knob of the entire IT stack
of the database for performance maximization. In contrast to
these offline tuning strategies, ONLINETUNE [1] offers an
online approach, using contextual Bayesian optimization for
adaptive database tuning in ever-changing cloud environments.
The widely utilization of Gaussian processes [16] in database
knob tuning is attributed to their theoretical capability to
balance exploration and exploitation.
Reinforcement Learning-based Tuning. Unlike OtterTune,
which segments the tuning process into various phases and re-
lays the optimal solution from one stage to the next, CDBTune
[5] introduces a more unified, end-to-end solution. Utilizing
reinforcement learning for database knob tuning, CDBTune
employs DDPG [17] as an agent, with the knob value as the
action, the database as the environment, the internal state of
the database as the state, and changes in database performance
as the reward. On the other hand, QTune [6] also incorporates
reinforcement learning but with a unique perspective, consid-
ering query statements during model training. It allows for
multi-level tuning, including query-level, workload-level, and
2
https://github.com/major/MySQLTuner-perl
3
https://pgtune.leopard.in.ua/
cluster-level adjustments. Typically, trained models struggle
to adapt to new tuning scenarios, necessitating a cold start.
Addressing this cold start problem, HUNTER [7] proposes a
solution that integrates genetic algorithms with reinforcement
learning. This method minimizes model training time by
independently performing various configurations on multiple
replicated database instances.
At present, there are some other studies related to database
knob tuning. LlamaTune [18] focuses on enhancing the sam-
pling efficiency of existing optimizers. Studies such as [19]
and [20] propose methods for extracting tuning rules from
text data. ReIM [21] adopts an empirically-driven white-box
method to tune the memory resource allocation in Spark [22]–
[24]. [25] utilizes the Plackett-Burman experimental design
approach [26] to rank database knobs by importance. [27]
concludes, based on relevant experiments, that database knob
tuning often requires adjusting only a few knobs. However,
it does not provide guidance on how to rapidly identify
these crucial knobs in a specific scenario. [28] performs a
detailed experimental comparison of relevant tuning tools
(e.g., OtterTune and CDBTune) in a real scenario. In a study
outlined in [29], the three key aspects of database knob tuning
(knob selection, configuration optimization, and knowledge
transferring) are experimentally compared, with SHAP [30],
SMAC [31], and RGPE [32] identified as the best algorithms
for these aspects, respectively.
III. O
VERVIEW OF OBTUNE
Domain Knowledge. Shallow domain knowledge such as the
knob’s value range and how to split the value range for
efficient sampling has been used in auto knob tuning [18],
[33]. In this work, OBTune is designed to integrate deep
domain knowledge. Our motivation is to help DBAs optionally
contribute domain knowledge regarding database functionali-
ties. While OBTune can achieve commendable tuning results
without this input, the inclusion of DBA insights enables even
better outcomes. Importantly, OBTune uses domain knowledge
to selectively tune knobs related to active functionalities,
enhancing tuning effectiveness and reducing potential risks.
This methodology caters to varying DBA expertise levels,
maximizing the use of available knowledge.
Knob Classification. Knobs in OBTune are primarily classi-
fied into two categories: functionality knobs and main knobs.
Specifically, if the adjustment of knob x directly affects the
performance of triggered functionality A, and the change
of knob x has no impact on the database performance if
functionality A is not triggered. Then x is classified to the
knobs of functionality A. For knobs that simultaneously affect
the performance of multiple functionalities, OBTune’s strategy
is to classify such knobs as main knobs. Furthermore, if a
tuning knob does not belong to any specific functionalities, it
also is classified to the main knob.
Definition 1. Direct metrics, that are used to directly reflect
the performance of a database functionality. For instance, the
direct metric for load balancing functionality in OceanBase
85
Authorized licensed use limited to: Zhejiang Tmall Technology Co.Ltd.. Downloaded on August 01,2024 at 03:39:58 UTC from IEEE Xplore. Restrictions apply.
BBAAD9C20180234D78A0072836F0B380F2B9B2091CE87BA0AFD98A34B1BC2BE43B4DB4389156AB0422B920089846DFEB92E921BAC1D0BB511BBFC261763E3FD6241121AD5E26F9F76406289767B74B475DC7EC897D66C41F589A219C63D0ACC8D7E62A964E3
相关文档
评论